AI in Fintech: What’s Really Changing (and Why It Matters Now)
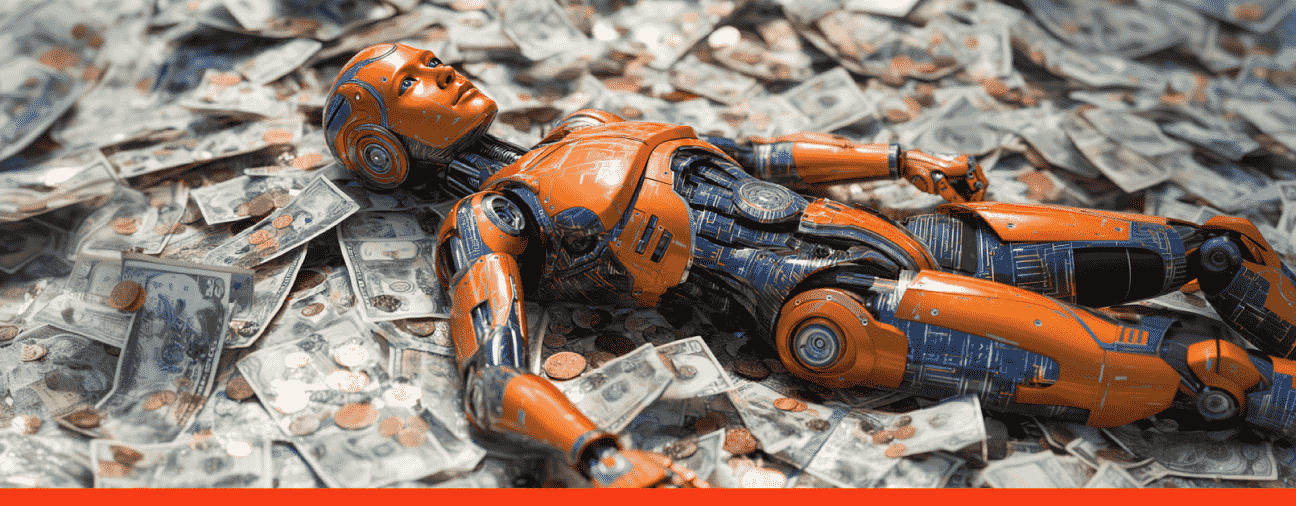
Over the past decade, artificial intelligence has quietly threaded itself into the DNA of fintech. AI is now powering high-stakes decision-making, underwriting billions in loans, flagging fraudulent transactions before they hit the ledger, and shaping the customer journey in real time. And yet, most conversations about AI in fintech still hover between cautious curiosity and abstract optimism. The truth is, the conversation has moved on. What used to be experimental is now operational. What was once a differentiator is fast becoming table stakes. The question facing fintech leaders today isn’t “Should we use AI?” It’s: “Are we using AI in fintech in the right way?”
This article isn’t a hype piece about AI or a collection of inflated promises. As a company that provides AI software development services, we aim to offer an informed look at AI in fintech how it’s quietly reshaping the fintech sector, not in a distant future, but here and now, and what that means for those building, buying, or scaling the next generation of financial products.
Adoption that is no longer hypothetical
Despite the complexity of implementation and the regulatory pressure that shadows every innovation in financial services, AI adoption in fintech isn’t slowing down; it’s increasing. Many companies have moved past pilots and proofs of concept. AI is now part of the operational core for an increasing number of players. For those still asking what is AI fintech, the answer is no longer theoretical; it’s the practical application of AI to optimize decisions, improve experiences, and drive efficiency across financial products and services.
What’s fueling this shift isn’t just the technology itself; it’s a combination of market dynamics, infrastructure maturity, and competitive urgency. A few key forces are driving real adoption at scale:
- Data advantage. Fintech platforms sit on a goldmine of behavioral, transactional, and contextual data, often in real time. AI thrives in these environments, especially when integrated into product flows like risk scoring, personalization, or fraud detection.
- Margin pressure. With rising customer acquisition costs and regulatory overhead, fintechs are under pressure to automate and optimize. AI offers a way to cut costs without worsening service quality, and instead, by automating tasks that used to require entire teams.
- Competitive pressure. The fastest-growing fintechs are deploying AI at scale: not just in products and operations but in areas like fraud detection, credit risk assessment, and compliance. This raises expectations across the board. Falling behind doesn’t just slow innovation; it risks signaling stagnation to users, investors, and regulators in a market where speed and adaptability are everything.
- Maturity of the tooling. From MLOps platforms to explainability frameworks and pre-trained financial LLMs, the ecosystem around AI has matured significantly. What used to require deep R&D investment can now be deployed with far lower friction.
- Regulatory readiness. As regulators become more fluent in AI risks and standards (with initiatives like the EU AI Act and the Fed’s guidance on model risk management), fintechs are gaining clearer rules to follow. This makes it safer to invest in AI at scale without risking compliance issues.
Together, these forces have pushed AI from theoretical potential into practical deployment. What was once a forward-looking investment is now a competitive necessity, and the following numbers reflect that shift.
- According to the FCA Artificial Intelligence and Machine Learning 2024 survey, 75% of financial institutions have already deployed AI.
- According to Statista report, AI spending in the financial sector is projected to grow from $35 billion in 2023 to $126.4 billion by 2028, reflecting a 29% compound annual growth rate (CAGR). This signals rapidly rising confidence in AI as a strategic investment across the industry.
- According to a PYMNTS report, finance leaders are leveraging AI in fraud detection (64%), risk management (64%), investment management (57%), and automation (52%), while 42% of chief experience officers see AI as a key enabler for improving onboarding and customer experience.
- Notably, 76% of financial services companies have launched AI initiatives, targeting both cost efficiency and revenue growth, with Wall Street firms increasingly investing in proprietary LLMs and high-risk domains like fraud prevention and wealth management.
The data paints a clear picture: AI is no longer a forward-looking initiative in fintech — it’s already reshaping operations, product strategies, and customer engagement across the board. From fraud detection and risk management to wealth advisory and onboarding, adoption is both broad and business-critical.
Where AI is already working and what that tells us
From Tier 1 banks to lean digital-first lenders, AI is being used to improve decision quality, reduce fraud, increase automation, and deliver more nuanced, responsive customer experiences. But more telling than where it’s used is how is AI used in fintech? The most effective applications don’t look like AI initiatives; they’re operational systems that blend into the core product architecture.
Here are six fintech AI use cases showing where AI is already reshaping the business of fintech.
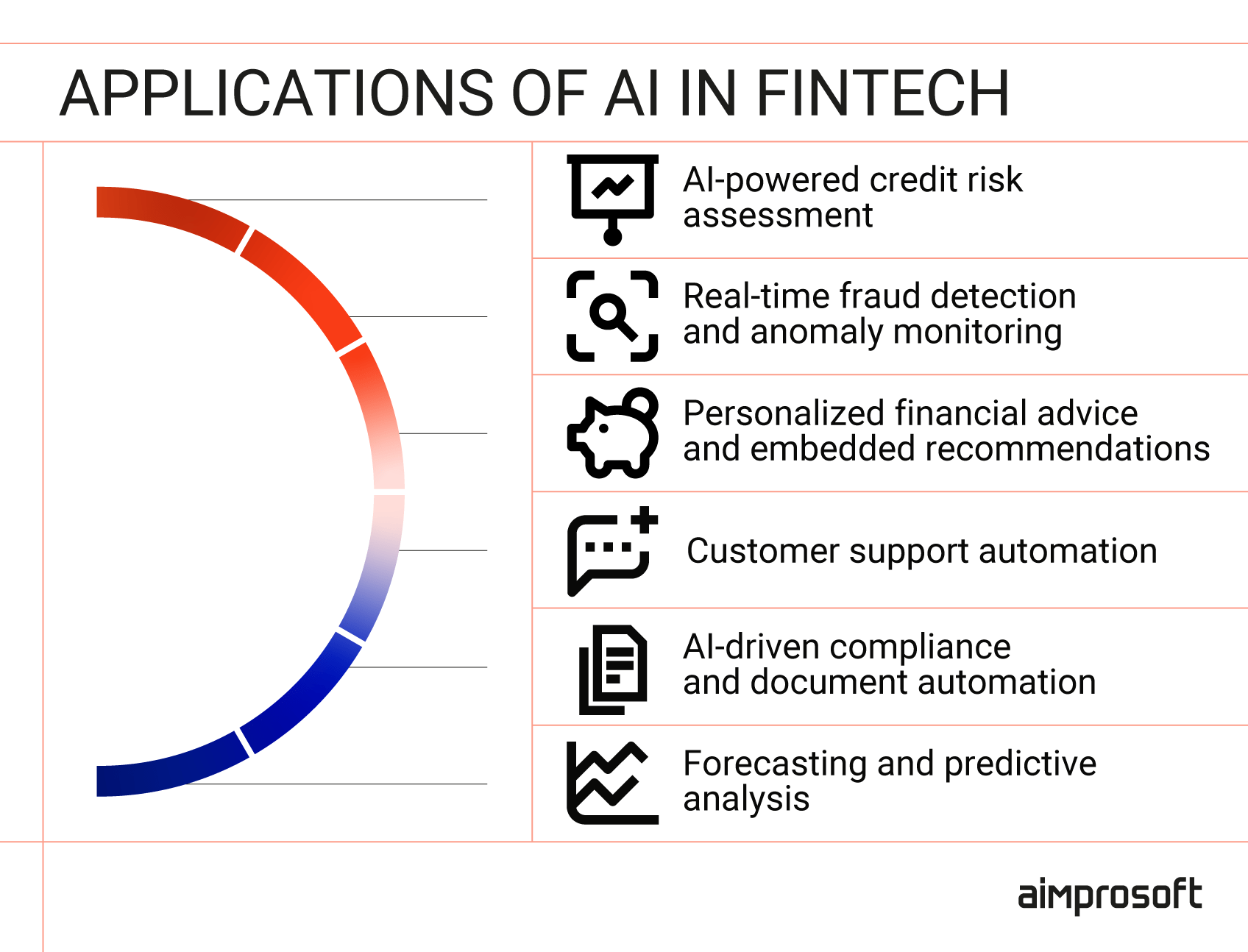
Key applications of Ai in fintech
AI-powered credit risk assessment
AI models in credit risk go far beyond traditional scoring. Machine learning algorithms are trained on vast and diverse datasets (including alternative data like transaction patterns, mobile usage behavior, and even social signals) to assess borrower reliability in real time. Unlike static rule-based systems, AI models continuously learn and adjust based on user activity, enabling more nuanced credit decisions, especially for thin-file or underbanked segments. They’re embedded directly into underwriting pipelines and lending workflows, making them integral to real-time decision-making at scale.
Real-time fraud detection and anomaly monitoring
AI is applied to fraud detection through a combination of supervised and unsupervised learning techniques. Models analyze transaction data, geolocation, device metadata, and behavior sequences to flag anomalies that deviate from normal user patterns — often before traditional rule engines would catch them. Neural networks and anomaly detection algorithms are trained on historical fraud cases and continuously refined with new inputs, enabling faster identification of novel threats. These systems run continuously across live payment and login flows, updating risk scores with every new user interaction.
AI-driven personalization and embedded product recommendations
AI powers personalization in fintech by building detailed user profiles from structured and behavioral data, such as spending habits, financial goals, lifecycle events, and response to past offers. Recommendation engines use clustering, collaborative filtering, and reinforcement learning to surface relevant financial products (loans, insurance, credit line increases) directly within apps or partner platforms. In embedded finance, these engines are deployed as APIs or microservices that trigger recommendations at contextually relevant touchpoints, during checkout, after major transactions, etc.
Conversational AI and customer support automation
Modern AI-powered support systems rely on NLP and LLMs fine-tuned to industry-specific documentation, FAQs, and interaction logs. These systems classify intent, extract entities, and generate human-like responses — often using retrieval-augmented generation (RAG) to pull up-to-date data from internal sources like help centers or CRM notes. They’re deployed via chatbots, voice assistants, or in-app messaging, with fallback logic and escalation flows when confidence thresholds drop. Unlike early-gen bots, these systems can handle nuanced financial queries and compliance-sensitive responses.
AI-enabled compliance and document automation
In compliance workflows, AI is used to parse and extract data from structured and unstructured documents, such as KYC forms, regulatory disclosures, or transaction records, using OCR and NLP techniques. Machine learning models flag inconsistencies, match names across sanction lists, and identify PEPs or high-risk entities. These tools integrate directly with onboarding flows or AML systems, providing real-time validation and risk scoring. Many platforms now embed AI into internal compliance dashboards for continuous monitoring, significantly reducing manual review cycles.
Forecasting and predictive analytics
AI is used in forecasting to analyze historical and real-time financial data, such as account balances, payment cycles, market behavior, or macroeconomic indicators, to predict future outcomes. In the context of AI in payments & fintech, these models help optimize everything from cash flow management and fraud detection to customer retention and investment strategies.
Models range from classic time-series algorithms to deep learning-based predictive pipelines that factor in seasonality, sentiment analysis, and external variables. These predictions are used in everything from cash flow projections for SMBs to customer churn modeling or trading signal generation. Deployed as part of analytics stacks, these models often power dashboards, alerts, and risk simulations across internal and external user-facing tools.
Want to learn more about AI and its impact on businesses? Explore adoption challenges, success stories, and practical insights on AI adoption to inform your next strategic move.
These six AI applications in fintech aren’t experiments; they’re already embedded in the operating models of fintech players across the globe. But while the current landscape offers plenty of proven value, it’s the next wave of AI adoption: from generative models in financial planning to autonomous systems in compliance that will reshape the competitive edge. Let’s take a closer look at where the momentum is heading.
The business case for AI in fintech: measurable advantages, not theoretical gains
While the technical advancements behind AI and fintech are impressive, the justification for adopting it isn’t just about keeping up with innovation; it’s about unlocking real, repeatable business value. For fintech companies operating in crowded markets, under scrutiny from both investors and regulators, AI is increasingly a tool of strategic leverage. The benefits of AI in financial services go far beyond automation. They enable smarter decisions, stronger compliance, and more personalized customer experiences.
Here’s how the most effective fintech organizations are turning AI into a business advantage.
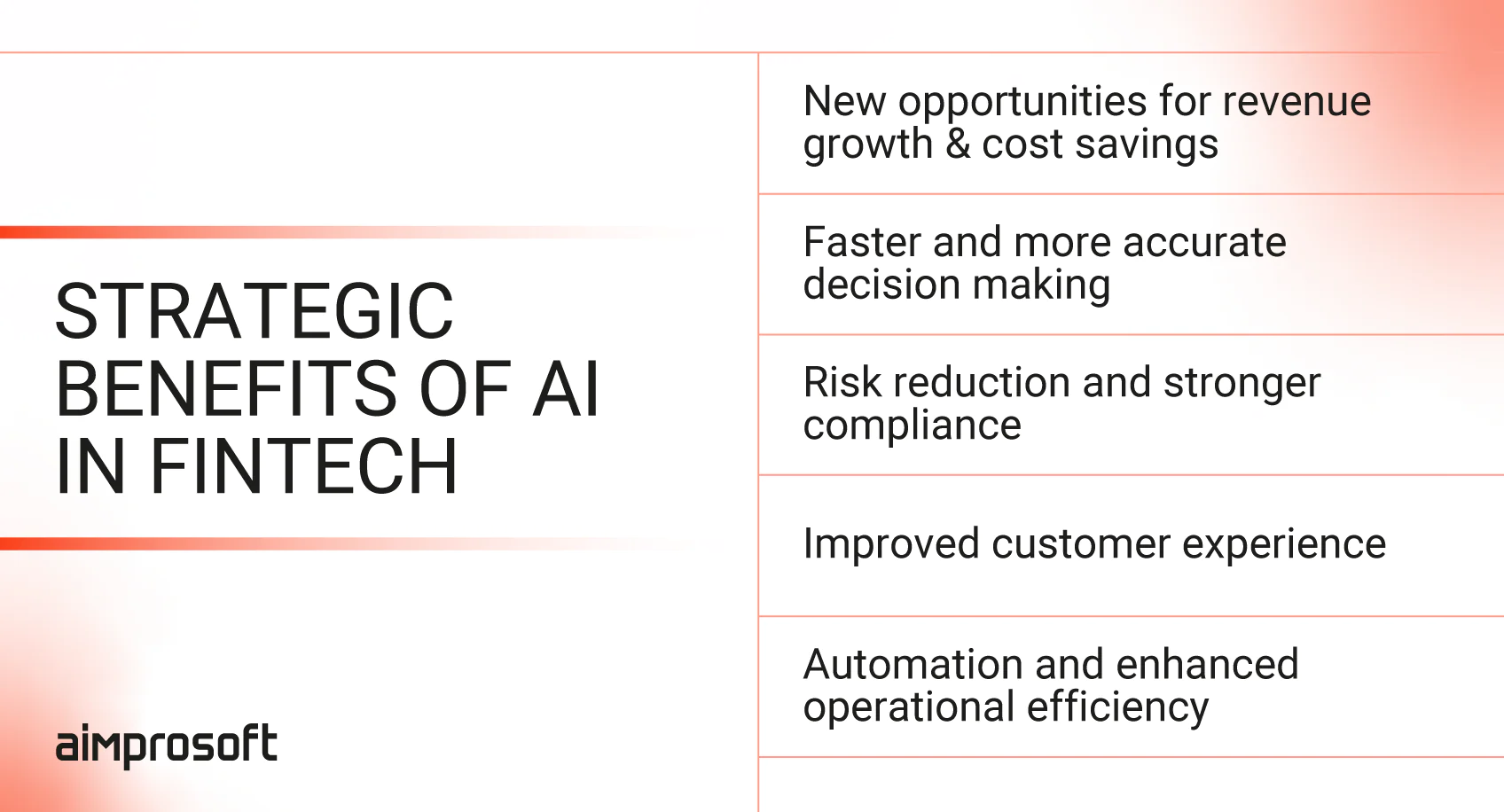
Strategic benefits of AI in fintech
1. Revenue expansion and cost reduction
Fintech companies are using AI to uncover new revenue streams through hyper-personalized product offerings, dynamic pricing, and real-time cross-sell/upsell recommendations. For example, machine learning models can analyze user behavior to tailor financial advice or trigger contextual offers, increasing conversion rates and customer lifetime value.
At the same time, AI-powered automation reduces the need for manual processes in areas like underwriting, fraud detection, customer support, and compliance — slashing operational costs without sacrificing accuracy. From AI chatbots reducing support overhead to automated KYC and AML checks cutting hours of manual review, the cost-saving potential is both immediate and scalable.
2. Faster decision-making
Artificial intelligence in fintech enables companies to process and interpret vast streams of financial data in real time — from user behavior and transaction patterns to credit scores and market fluctuations. This speed translates into faster decisions in critical areas like loan approvals, risk assessment, fraud detection, and investment management. For example, an AI-driven lending platform can evaluate a borrower’s risk profile and return a credit decision in seconds, significantly reducing customer drop-off and operational delays.
Beyond individual transactions, AI supports strategic decision-making by identifying trends, forecasting demand, and simulating different financial scenarios. Teams no longer need to wait for end-of-month reports — AI-powered dashboards and predictive analytics give them live insights, allowing product leaders, analysts, and executives to adapt quickly to changes in user behavior, market dynamics, or regulatory pressure. This real-time visibility helps organizations stay ahead, not just keep up.
3. Risk reduction and stronger compliance
AI helps fintech companies proactively manage risk by continuously monitoring transactions, user behavior, and external market signals. Machine learning models can detect fraud patterns, flag suspicious activity, and assess creditworthiness far more accurately than traditional rule-based systems. This not only reduces financial losses but also minimizes false positives — enabling faster, more accurate decision-making across fraud prevention, credit scoring, and insurance underwriting.
On the compliance side, AI streamlines time-consuming tasks like KYC verification, transaction monitoring, and regulatory reporting. Natural language processing (NLP) can even analyze new regulatory documents to flag relevant changes automatically. By embedding AI into compliance workflows, fintechs reduce the cost and human error associated with manual checks — all while staying audit-ready and aligned with evolving regulations like GDPR, PSD2, and AML directives.
4. Improved customer experience
AI in financial technology enables companies to deliver tailored, intuitive user experiences at scale. By analyzing user behavior, financial habits, and preferences, AI models help personalize product offerings, recommend relevant financial services, and provide real-time insights that feel human. This results in higher engagement, stronger retention, and greater lifetime value.
At the same time, AI-powered chatbots and virtual assistants ensure 24/7 support without overloading human teams. These tools can resolve common queries, guide users through complex tasks like onboarding or loan applications, and escalate issues only when necessary — reducing wait times and boosting customer satisfaction.
5. Automation and enhanced operational efficiency across teams
AI-driven automation eliminates repetitive, manual work across back-office and customer-facing processes. Tasks like transaction categorization, claims processing, data validation, and document handling are executed faster and more accurately by machine learning models, freeing up teams to focus on strategic, high-value activities.
In operational terms, this means faster turnaround times, fewer errors, and significantly lower cost per task. Whether it’s streamlining internal workflows or accelerating user-facing interactions, AI allows fintechs to scale without linearly increasing headcount or overhead — a critical advantage in a margin-sensitive, fast-moving industry.
Our team helps fintech companies turn AI from a buzzword into a bottom-line advantage — whether it’s smarter automation, better risk modeling, or hyper-personalized user experiences. Tell us what you’re building, and we’ll help you shape the strategy and team to get there.
CONTACT USAs you can see, the benefits of AI in financial technology sector are not abstract. They’re already shaping how modern financial platforms are built, scaled, and defended. But they only become possible when AI is integrated with intent, namely, aligned with business goals, supported by the right infrastructure, and executed by teams who understand both the technical and regulatory terrain.
Real-world AI use cases in the fintech industry
Across fintech and adjacent financial services, AI fintech is no longer a speculative play; it’s delivering measurable outcomes tied to revenue growth, cost savings, and operational scale. The benefits of AI in finance are becoming increasingly clear as more companies move beyond experimentation and achieve tangible business impact through AI integration. Below are select cases demonstrating that shift.
1. PayPal: using AI to drive conversion, personalization, and post-purchase engagement
PayPal is leveraging AI not just for fraud detection, but to create new growth levers for businesses using its platform. Through a suite of AI-driven demand and conversion tools, PayPal helps merchants:
- Attract and engage new customers
- Enable fast, low-friction logins and guest checkouts
- Retarget shoppers with personalized recommendations and post-purchase offers
One standout feature is AI-powered smart receipts, which personalize follow-up communications with tailored product suggestions and cashback deals. With nearly 45% of PayPal users opening their receipts, this functionality gives merchants a powerful way to re-engage customers and drive repeat purchases — turning a routine confirmation email into a revenue-generating channel.
By analyzing shopper behavior and applying insights across its vast network, PayPal gives even small merchants access to the kind of AI-driven personalization typically reserved for global retailers.
2. Upstart: redefining creditworthiness with AI-powered underwriting
Upstart is an AI-driven lending platform that helps banks and credit unions offer more inclusive and precise credit decisions. Its machine learning models evaluate borrowers using alternative data, such as education, employment history, and account activity, to predict default risk more accurately than traditional FICO-based systems. As a result, Upstart has approved 27% more applicants at 16% lower interest rates, delivering over $7.8 billion in personal loans to 620,000+ borrowers across all demographics.
Beyond its own lending platform, Upstart offers financial institutions access to its AI through a white-label solution and a standalone Credit Decision API. These tools allow banks to plug into Upstart’s underwriting technology and integrate smarter credit decisioning into their existing workflows without overhauling internal systems.
3. Klarna: scaling customer service and personalization with AI-powered assistants
Klarna, a global fintech platform with over 85 million active users and 2.5 million daily transactions, is redefining customer experience through AI. Its flagship AI Assistant, built with LangGraph and LangSmith in collaboration with OpenAI, now handles two-thirds of all customer chats across 23 markets, automating over 70% of repetitive support tasks. In less than a year, it has conducted 2.3 million conversations, performed the work of 700 full-time agents, and reduced average resolution times from 11 minutes to under 2, while maintaining human-level customer satisfaction.
Beyond support automation, Klarna uses AI for root cause identification, reducing repeat inquiries by 25%, and delivering tailored recommendations post-purchase. This combination of instant response, 24/7 multilingual availability (35+ languages), and predictive personalization has made the AI Assistant a driver of both efficiency and loyalty. It’s also projected to contribute $40 million in profit improvement in 2024 — a clear signal of how deeply AI is integrated into Klarna’s operational and strategic model.
4. JPMorgan: automating legal work and scaling AI across the enterprise
JPMorgan, the largest bank in the U.S., has been steadily integrating AI across legal, operational, and customer-facing workflows. Its proprietary COiN platform (Contract Intelligence) automates the review of commercial credit agreements, a task that previously required 360,000 hours of legal work annually. By using machine learning to extract key clauses, assess risk, and ensure compliance, COiN can now analyze 12,000+ loan agreements in seconds, significantly improving accuracy and freeing legal teams to focus on high-value strategy and advisory work.
Beyond COiN, JPMorgan has launched the LLM Suite, a generative AI toolkit available to thousands of employees, and deployed AI tools in call centers to support Chase Bank customers in real time. The firm’s investment in tools like IndexGPT, an AI-powered product for building personalized investment strategies, signals a broader shift toward embedding AI into core business functions. With initiatives led by the Chief Data and Analytics Officer, JPMorgan is positioning AI not as an add-on, but as foundational to how its 300,000+ employees operate and compete at scale.
5. Ant Group (Alipay): real-time credit risk assessment at scale
Ant Group, the fintech powerhouse behind Alipay, applies AI at an unparalleled scale, serving over 1 billion users with real-time credit evaluations, lifestyle tools, and embedded financial services. Its Zhima Credit system uses structured and unstructured behavioral data — from payment history to e-commerce activity, to instantly assess creditworthiness and underwrite microloans within seconds, with delinquency rates kept consistently below industry benchmarks. Behind the scenes, Ant’s AI infrastructure runs over 50,000 risk model iterations daily, powering one of the most efficient and inclusive lending ecosystems in the world.
Now expanding beyond finance, Ant has launched Zhixiaobao, an AI-powered virtual life assistant integrated into Alipay. Built on Ant’s proprietary Bailing foundation model, Zhixiaobao helps users handle daily tasks, from hailing taxis and ordering meals to managing entertainment and travel, through intuitive voice or text prompts. Features like AI-powered image search and translation (via the new Tanyixia function) further cement Alipay’s evolution into an AI-native “super app,” where financial services, lifestyle management, and personalization converge in a single interface.
We help fintech companies apply the same principles — but tailored to your product, team, and goals. Whether you’re scaling an existing platform or starting something new, our experts can help you build with AI the smart way.
CONTACT USThese examples share a common thread: the AI in fintech initiatives were strategically embedded into core systems, aligned with business KPIs, and built to scale. Whether in customer-facing tools or internal efficiencies, fintech AI is proving its value when tied to clearly defined outcomes — not experimentation for experimentation’s sake.
What’s next: emerging AI trends shaping fintech’s evolution
AI for fintech adoption may be accelerating across fintech, but it’s not happening uniformly; it’s coalescing around specific areas where the pressure to scale, personalize, and comply is greatest. The most transformative shifts aren’t coming from headline-making models or novelty features; they’re emerging where AI meets product strategy, infrastructure, and regulation.
Below are five trends redefining how financial technology is being built and deployed. For business leaders, understanding these shifts isn’t optional; it’s essential for shaping relevant, scalable, and future-proof fintech products, especially as the future of AI in finance continues to accelerate innovation and reshape expectations.
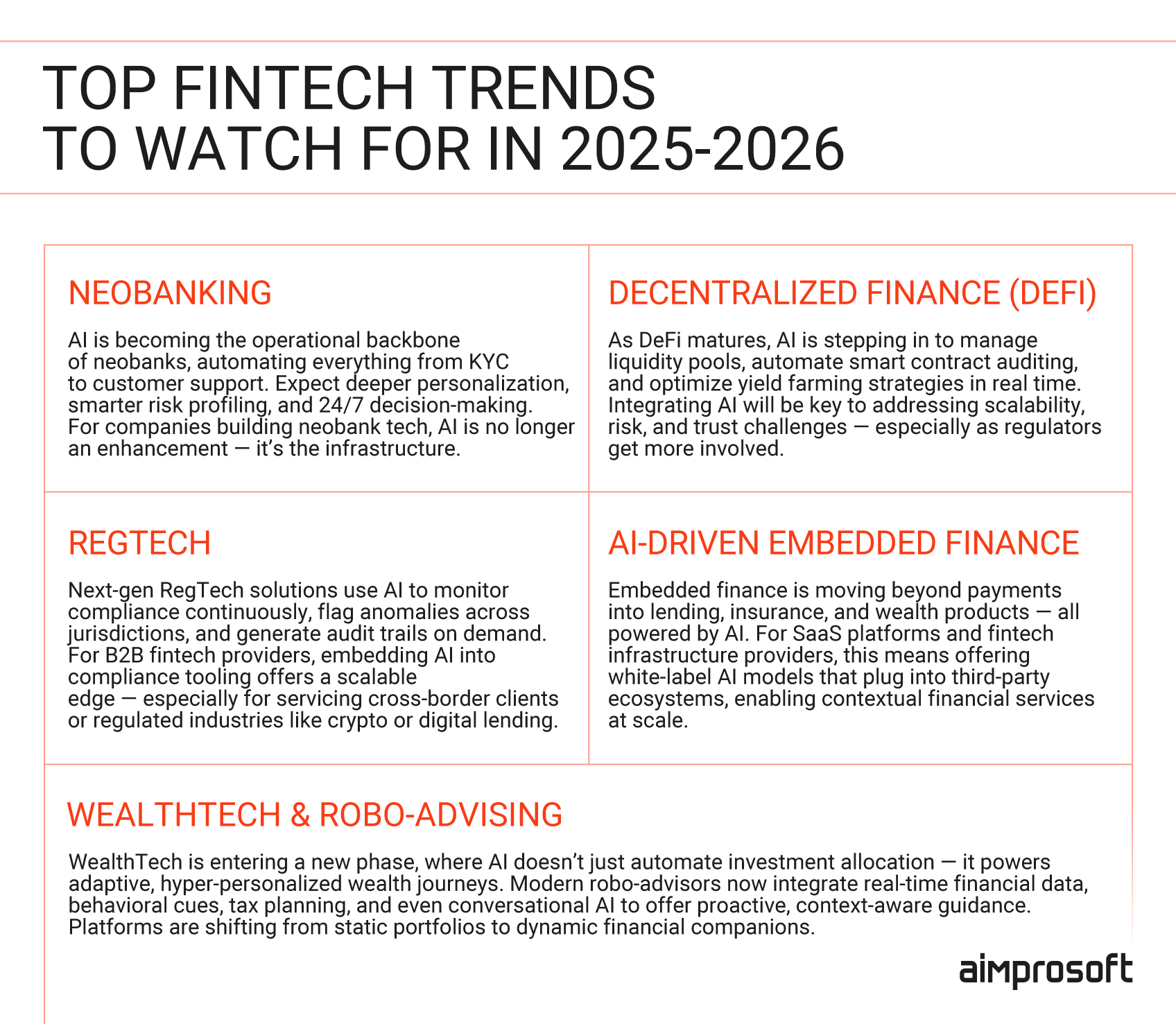
Top fintech trends to watch for in 2025-2026
1. AI-powered neobanking: where automation becomes infrastructure
Neobanks were built to bypass the inefficiencies of traditional banking, and AI is now the engine that allows them to scale without adding headcount. From real-time KYC and credit scoring to conversational support agents that learn over time, AI enables neobanks to deliver full-service banking experiences with leaner teams and faster responsiveness.
But the real value is deeper: AI enables contextual decision-making, recommending personalized products, adjusting risk profiles based on behavior, and detecting fraud before it happens. For companies building neobank platforms or integrating digital banking services, AI is no longer an enhancement layer. It’s the operating system.
2. DeFi + AI: building intelligent, self-optimizing financial ecosystems
Decentralized finance has already challenged legacy financial infrastructure; now, AI is stepping in to address the operational complexity that’s limiting DeFi’s next phase. Smart contract auditing, liquidity pool optimization, and market anomaly detection are being automated using machine learning, reducing reliance on human oversight in real-time environments. As DeFi matures and regulatory frameworks tighten, integrating AI becomes essential for building resilient, trustworthy protocols.
3. RegTech: scaling compliance without scaling costs
Compliance is a constant friction point in fintech, especially for products that span jurisdictions, serve regulated industries, or handle sensitive data. RegTech AI solutions for fintech are changing that dynamic by continuously monitoring transactions, scanning for irregularities, and generating detailed audit trails, all in real time.
For fintechs, serving institutional clients or operating in multi-market environments, embedding AI into compliance processes isn’t just efficient. It’s a growth enabler. Scalable compliance means faster go-to-market timelines, lower legal risk, and a stronger foundation for long-term partnerships with banks, insurers, and governments.
4. AI-driven embedded finance: financial products, precisely when and where users need them
The embedded finance boom has moved beyond checkout lending and payment gateways. Today, AI is powering context-aware financial experiences across industries, surfacing the right product (loan, insurance, investment) at the exact moment a user shows intent, risk profile, or behavioral readiness. Businesses that can integrate AI-based underwriting, dynamic pricing, and customer segmentation into their offerings will unlock new revenue streams while delivering frictionless value to users.
5. WealthTech and robo-advising: building intelligent, personalized financial journeys
WealthTech is moving far beyond static robo-advisors. The new generation of AI-driven platforms is capable of adjusting portfolios in real time, generating goal-based recommendations, and providing predictive insights based on behavioral and transactional patterns, often through conversational interfaces.
This isn’t just about access. It’s about intelligent financial enablement. Fintech companies that want to offer investment or advisory services need to think in terms of adaptive financial journeys — experiences that evolve with the user, offer transparency, and meet compliance from day one. AI is what makes that possible at scale.
Why these trends matter now
Individually, each of these trends represents a specialized domain of innovation. But together, they point to a larger reality: fintech is becoming AI-native by design — not just in feature sets, but in business models, architecture, and customer value delivery. For product owners, founders, and platform leaders, the takeaway is clear: the future of AI in fintech won’t be defined by those who adopt AI the fastest but by those who understand where and how AI is used in finance and how it creates the most leverage.
Wrapping up
AI in fintech is no longer a side experiment or innovation badge. It’s the connective tissue powering the next generation of financial services. The companies seeing the greatest return aren’t those chasing novelty but those approaching fintech solutions development with AI deliberately, aligning it with clear business goals, scalable infrastructure, and a deep understanding of the regulatory nuances that define this industry.
Whether you’re building a new product, expanding into new markets, or optimizing existing operations, the question isn’t whether to adopt AI; it’s how to do it in a way that creates real, measurable impact. If you’re ready to explore how AI can create strategic value for your fintech business, get in touch with our team.
FAQ
How do we know if our fintech product is ready for AI integration?
AI readiness isn’t just about data. It’s about infrastructure, organizational alignment, and having a clear use case. If you already collect structured and behavioral data, have real-time user interactions, and face bottlenecks in decision-making, chances are you’re sitting on high-value AI opportunities. The key is to start with a well-scoped, measurable initiative. We often recommend an AI readiness assessment that covers data quality, team capacity, tech stack compatibility, and regulatory constraints. This reduces risk and prevents wasted investment on models that can’t be productized.
Can we still use AI effectively if we operate in a highly regulated space?
Yes, you can. In fact, many of the most advanced AI use cases in fintech (like underwriting or fraud detection) exist in regulated environments. The difference lies in how you approach model explainability, governance, and traceability.
Working with experienced partners who understand financial compliance frameworks (like GDPR, PSD2, or the AI Act) is critical. From AI model documentation to human-in-the-loop design, responsible AI doesn’t slow innovation; it makes it scalable and defensible.
We’ve experimented with AI before, but it never scaled. What are we missing?
Many companies succeed with proofs of concept but hit a wall when trying to move from prototype to production. The issue often isn’t the model; it’s the lack of MLOps, data pipelines, versioning, and cross-team alignment needed to support AI at scale. Unlocking the benefits of AI in finance requires more than building a good model. It demands a system that can monitor performance, retrain over time, and integrate seamlessly into your product lifecycle. That’s where the right technical partner makes the difference.
Should we build an in-house AI team or work with an external partner?
It depends on your core business model, time-to-market pressures, and internal capacity. Building in-house offers long-term control — but it requires serious investment in data infrastructure, hiring niche talent (ML engineers, data scientists, MLOps experts), and ensuring cross-functional collaboration with product and compliance teams. That’s a tall order, especially if AI isn’t your core competency.
By outsourcing software development services to an experienced external partner you can accelerate time to value, de-risk early phases, and provide architectural guidance that supports long-term scalability — even if you later build your own team. Many fintechs take a hybrid approach: opting to outsource fintech AI development services and co-building with AI fintech companies who help them lay the technical and strategic groundwork, then gradually internalizing key capabilities over time.