Computer Vision in Healthcare: Revolutionizing Disease Identification to Human Doctor-Level in Medical Imaging
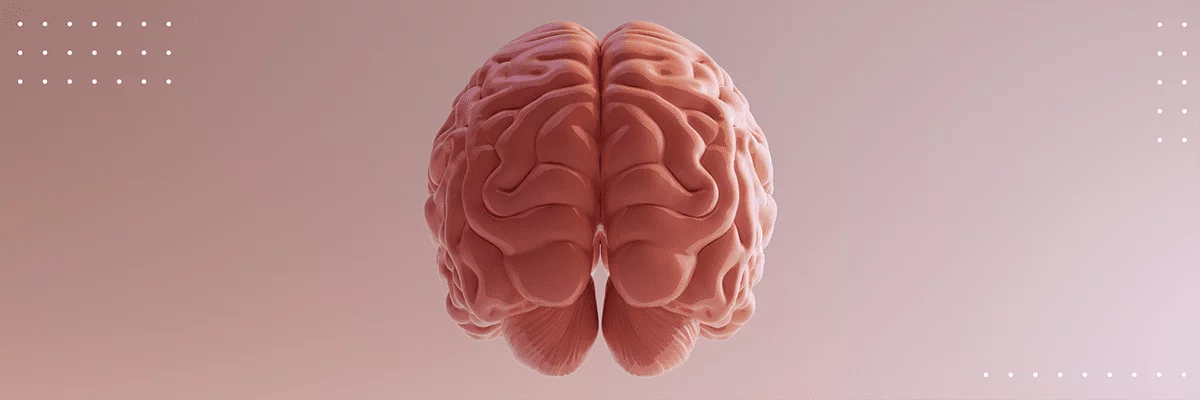
Key takeaways
- Machines can perform many tasks better than people backed by math and software. Further on, we’re going to uncover the capabilities of computational decision-making.
- The application of computer vision in the medical field has a lot of promise for capitalization. Learn what triggered a 200% growth of investments here.
- The evolution of machine vision touches on ethical concerns and shared responsibility among solution suppliers and consumers. Read on to learn more about compliance with regulatory guidance.
- You do not have to be tech-savvy to launch a product. Idea and data scientists are all you need to get things done. Read about a tech stack we use to implement computer vision.
- Aimprosoft’s experience in delivering AI-backed solutions to make a medical app.
With many tasks, a computer in healthcare with neural networks now works better than people. Of course, not so accurately as we wish, but without fatigue and the desire to escape from the daunting routine tasks.
That’s it. There has been an impressive breakthrough in the field of object recognition during the last decade. The improvements touched on both the basics of learning neural networks and architectural solutions.
For example, deep learning (an ML algorithms class that uses an appropriate multi-layer neural network) community developments have advanced in the accuracy of object recognition on open and private data sets from 70 to 98% accuracy.
Shocked?
Advances in medical vision technology for human health and medical application have been instrumental there, especially in the area of object recognition. An error rate of 3.5% comparatively to 5% of a human rate means that we’re at the point to pass on the reins to machines in object recognition.

Let’s explore why computer vision in healthcare applications is valuable as the technology for improving the performance of doctors and hospitals.
What is computer vision in healthcare?
Computer vision (or machine vision) is a branch of artificial intelligence that includes algorithms for detecting, tracking, and classifying visual objects, image depth estimation, instance and semantic segmentation, etc. Computer vision for healthcare innovation attempts to model the mechanism for receiving and processing visual information in the human brain.

We have experiences that we have had throughout our lives. Likewise, a neural network has a set of images that have been shown to it to explain certain general principles.
By using a set of methods, it gives the computer the ability to “see” and extract information from what it sees. To teach a computer to “see,” deep learning technologies are used. A lot of data is collected to highlight features and combinations of features for further identification of similar objects.
Image recognition is at the heart of the process that opens up a variety of opportunities for computer vision applications in healthcare.
The article goes on to deal with three interrelated issues of concern:
- Accurate medical diagnosis;
- Faster diagnoses and cure prescription;
- Treatment cost optimization for patients.
Let’s discuss what Aimprosoft can do for you with computer vision.
CONTACT USCommercialization perspective of AI-based diagnostics via application of computer vision
Why does healthcare need computer vision?
People tend to pay for addressing the plight of their living. Who could object to nip illness in the bud? It gives additional value in providing care services for consumers in medical settings.
As stated in the new findings, cancer and cardiovascular disease (CVD) are of greatest interest to many entities ready to invest and gain advantages. AI startups’ fundraising results strike with $2.2 billion since 2017, showing a 200% growth compared to the total since 2010.
It is expected that AI will not only accelerate physicians’ speed but also improve value-based care. If the doctor does the time-consuming work manually, it will take a lot of time. Most importantly, errors may occur because a person trite is tired. To avoid this, the neural network is employed as an adviser.
The Ministries of Health in many countries have already approved such software. This is already a significant market where computer vision techniques can speed up work and make analyses more accurate.
Look at these market demands in healthcare that can be met with computer-aided diagnosis systems based on machine learning algorithms.
Why does healthcare need computer vision?
Greater safety | Face recognition-based access to sensitive patients’ information improves control systems. |
Better service | Rapid facial identification shortens customer service time response and offers personalized services for patients. |
More strengthens human capabilities | Computer vision becomes a virtual doctor assistant that allows seeing things remaining unnoticed by the human eye. |
Faster performance of routine tasks | Compared to humans, a machine-based recognition might take a few seconds with pretty appropriate accuracy. |
Autonomy | Development of unmanned processes running tirelessly (for example, in robotic surgery) becomes possible. |
Billion-dollar applications of computer vision in medicine
In precision medicine, there are a large number of tasks associated with image processing, on which the doctor spends quite a lot of time. The list of improvements resulting from CV impresses. It heavily contributes to early disease detection, increased accuracy in medical image interpretation, and faster diagnosis, which leads to more affordable treatment.
Object detection is at the heart of such computer vision tasks as instance segmentation, image captioning, object tracking, and more. The following four tasks are defined as main in charge of CV for medical needs:
- Object recognition – e.g., cancerous skin moles’ detection through computer vision.
- Object detection – e.g., a lung disease abnormality detection through X-ray images.
- Semantic segmentation – e.g., detection and segmentation of polyps from colonoscopy videos.
- Instance segmentation – e.g., abdominal organ segmentation and recognition from CT scans.
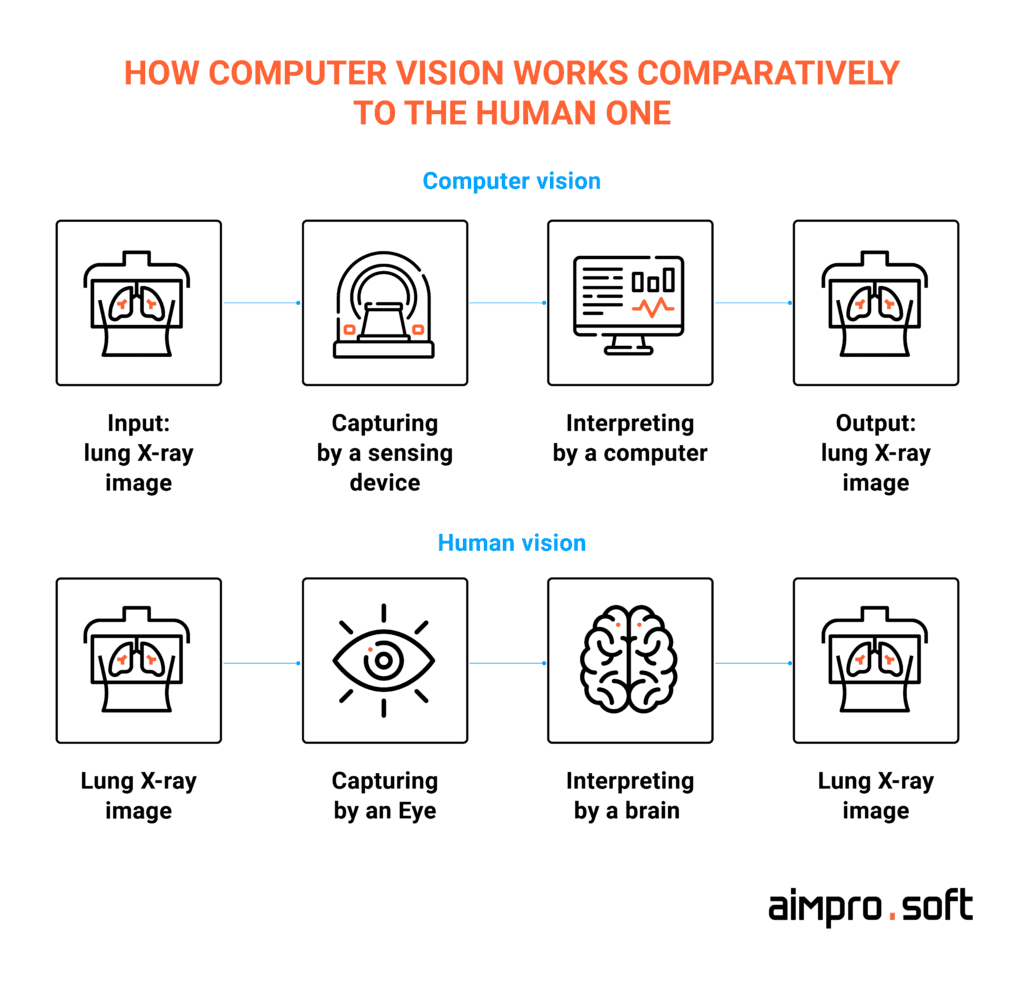
Computer applications in healthcare historically turned out to work with images in radiology because of the intention to see beyond human eye capabilities. The medical technology uses we’re going to go through encompass the most common cases (radiology) and other computer vision projects.
Human-computer collaboration with object recognition of cancer
According to the American Academy of Dermatology, skin cancer affects the most frequently among other cancer types in the USA, with almost 9 500 cases diagnosed every day. Early detection of pathology is needed to prevent malignant neoplasms among people, which is the key to successful treatment planning at early stages on the way to saving lots of lives.
Why is the perspective for ML here?
Deep convolutional neural networks (CNNs) and vision transformers are full of promise to cope with tasks related to fine-grained object categories. And this is exactly what an automated classification of skin lesions is. Since fine-grained variability is a specific feature of the skin lesions, a trained model can properly discover disease by pixels.
Here is an example of a simple step-by-step flow of skin cancer detection via image analysis.
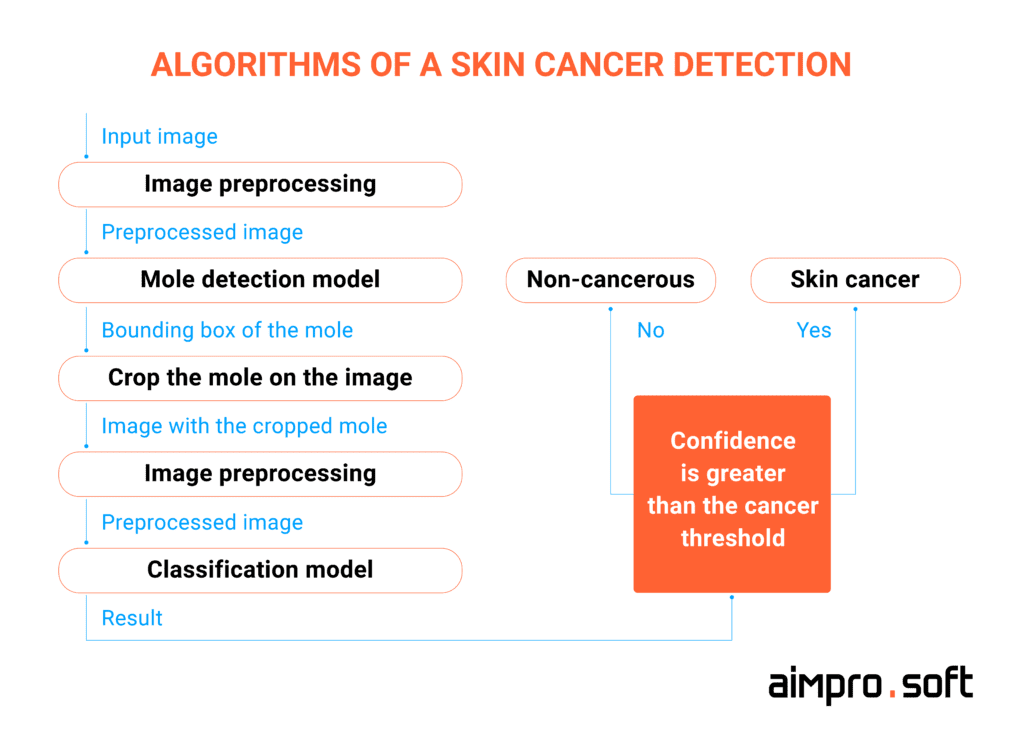
Here should be noted an AI-based mobile app Scanoma from Australia that can investigate skin blemishes for being malignant tumors, which is particularly relevant for people living in the sunniest areas.
25% of adults in the USA use at least one digital health app, which forces hospitals to incorporate mobile solutions in the care process. Read on how to build a healthcare app.
In the meanwhile, researchers from MIT, jointly with dermatologists, created a system able to distinguish suspicious pigmented lesions (SPLs) with over 90.3% sensitivity. Wide-field images captured by smartphones and a convolutional neural network (CNN) served as the basis for a dermatologist-level classification of cancer.
Abnormality detection in radiology
Touching on the pandemic rise, computer vision systems in the medical area sparkled with new colors. For example, many images of the lungs are taken. Therefore, the need to relieve the workload of doctors and speed up the processing of X-ray images has noticeably increased.
Computer vision is becoming a virtual doctor’s assistant here. CV can analyze medical images such as X-rays, MRIs, and ultrasounds, helping to improve the accuracy of diagnosing diseases.
Enter CheXNet by Stanford ML Group. They can detect pneumonia from chest X-rays by means of a 121-layer convolutional neural network. The accuracy of results exceeds practicing radiologists.
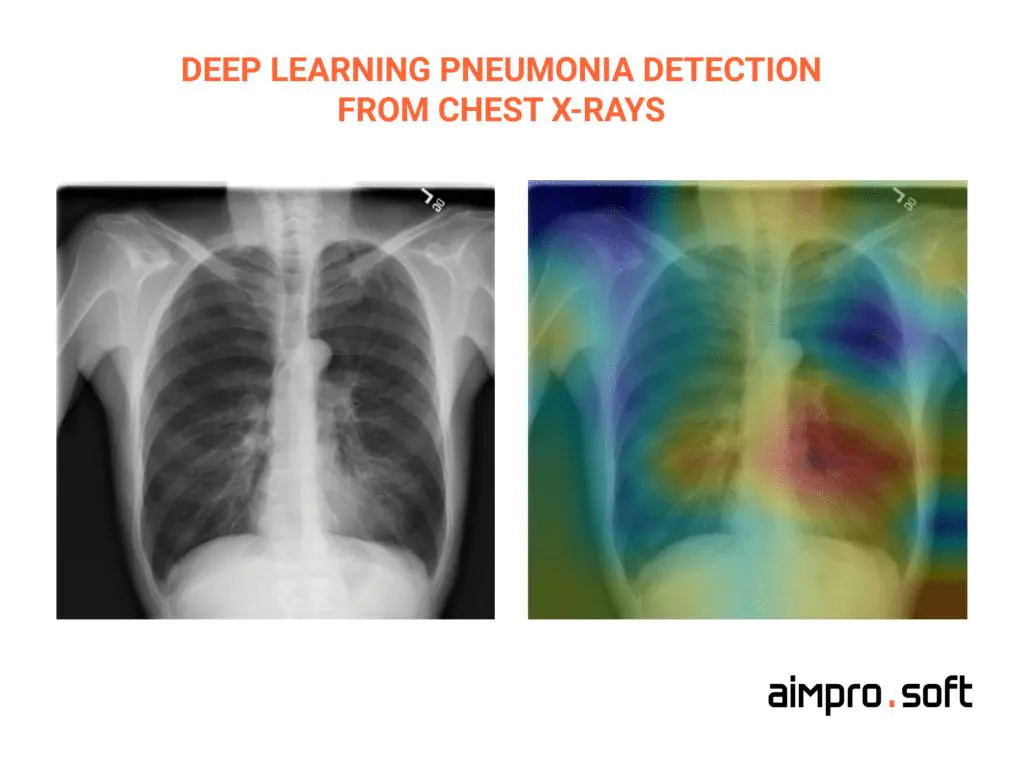
Another example, Microsoft InnerEye, advanced greatly in finding tumors on MRI images with high accuracy. Imagen used the potential of the largest dataset of medical images to improve interpretation by clinicians in the area of wrist bone fractures, as well as IBM Watson Imaging Clinical Review’s efforts in the same area to make patient care more affordable and accurate.
Up to 10% of radiation reduction is achieved due to the computer vision application while screening that accelerates diagnosis, reducing patients’ time spent in CT scans.
Neural network algorithms’ capability admired is the removal of unnecessary noise and distortion from X-rays and CT (computed tomography). This relieves patients from spending too much time in the CT scans, taking less radiation from the usual 25% to 10%. In addition to incalculable gains for health, the continuous development of AI-powered computers will lead to the total replacement of X-ray and CT machines. It’s a significant shift into non-radiation screening with immediate results.
Dermoscopic medical image segmentation
Image segmentation is one of the most valuable processes in medical imaging computer vision for the reason that it extracts a region of interest (ROI). How does it work?
A specified description is followed by dividing the image into areas based on the given description. A starting point could be to segment body organs or tissues in computer applications in the medical field for border detection, to detect or segment tumors. Thus, doctors will get an outcome as computer-aided separation of the region of interest (prospective pathology) and background.
Apart from white and black, there is a gray area on medical images.
In order to better recognize images with fuzzy borders, which is inherent in most medical images, fuzzy set theories and neutrosophic sets are used for segmentation during uncertainty handling. Look at Damae-Medical, they propose to see beyond appearance with their advanced solution for melanoma diagnostic.
Computer vision in surgical robotics: hype or hero
Surgical robotics that is likely soon to enter many operating rooms should not “work” without computer vision. Around 900,000 robot-assisted surgeries were performed in 2018 in the U.S.
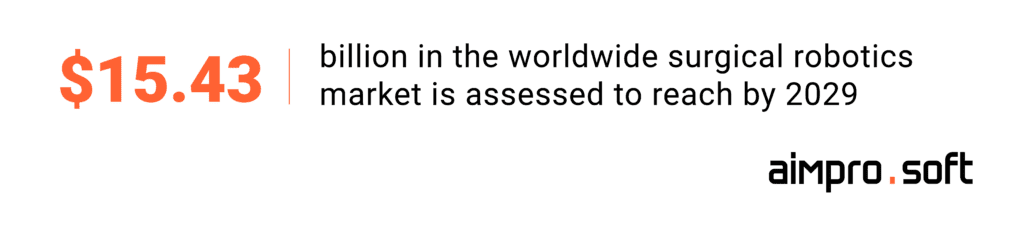
First, we are talking about surgical procedures that require tiny incisions that are difficult to make while holding instruments in human hands and maneuvering them. Trained computer vision is able to act like a human during an operation, immediately recognizing and responding to the changes. Despite so much precise jewelry work being here, traditional robotic surgery still implies the control of a conventional human surgeon who controls robotic hands.
Another case approves that the surgeon can plan how best to remove the tumor in advance. For example, during preoperative mapping, if to take fMRI scans (the sequence of 3D photographs of the brain to define active areas of the brain), surgeons can clearly find out that the tumor doesn’t intersect speech and motor centers in the human brain.
Computer and machine vision saves time and life here.
Activ Surgical, a Boston-based startup, is on the front line of reducing surgical complications concerned with blood loss and perfusion in real time by integrating enhanced computer vision, ML, and AI.
Let’s turn computer vision to your advantage.
CONTACT USBuilding an autonomous system for telemedicine
The coronavirus pandemic has brought a lot of harm but, at the same time, has become a strong impetus for the healthcare software development of telemedicine. How can recognition systems help here?
First of all, it is to carry out the primary computer-assisted diagnosis of some diseases by images without the need for an in-person doctor examination.
Doctor appointments can be booked remotely, which is a perfect solution for telehealth.
For example, for patients staying in rural areas, forcibly isolated because of pandemics, or having lost medical supervision, machine learning and computer vision solutions are going to be the way out in terms of replacing well-trained clinicians who, as usual, conduct examinations of patients.
Let’s take diabetic retinopathy (DR) and foot ulcers (DFU). They can be already diagnosed through computer vision for medical imaging analysis. The researchers and engineers from Sri Lanka went further by developing an Intelligent Diabetic Assistant that makes diagnoses and prioritizes treatment by observations taken from the screen autonomously with an 88.5 score, which is considered a pretty good result.
Computer vision in the medical field: contribution to cardiology
The non-invasive imaging methods in cardiology (CT, MRI, PET, SPECT, USG, etc.) have more potential to be enhanced with image analysis algorithms.
Time-consuming manual segregation of cardiac images with CT and MRI spurred the emergence of neural network-based segmentation. Has been applied in a wide variety of scenarios, to date, the computer vision medical applications encompass the following cardiology issues:
- visualization of coronary arteries (for detecting breast cancer, too);
- analysis of calcium in atherosclerotic plaques on computed tomography images;
- automatic image diagnostic for heart pathology detection;
- echocardiographic views analysis;
- cardiomyopathy diagnosis in nuclear cardiology;
- calculation of variables in cardiac MRI and more.
And illustrating disruptors here, HeartFlow is worth mentioning. They created a noninvasive test based on deep learning algorithms that build 3D models of the blood vessels that feed the heart. It allows avoiding the intrusion of a catheter for getting blood flow measurements. Now, they can be done with a lower risk for patients from taking measurements obtained from a CT scan.
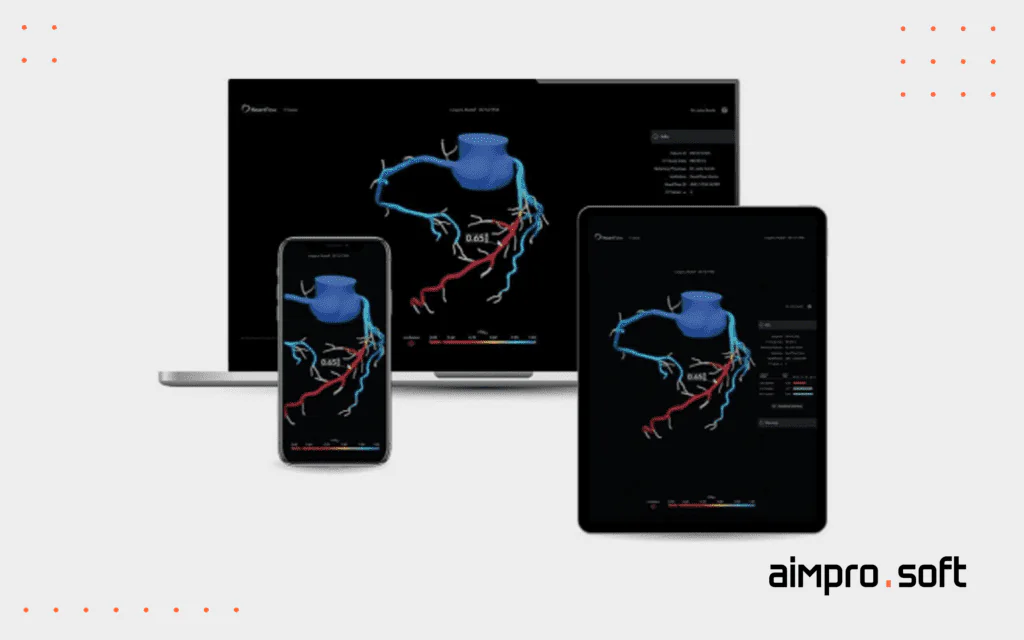
Machine vision applications allow labs out from overdrive
The application of computer vision in healthcare isn’t limited by image recognition, although it is considered undoubtedly the most popular employment.
The high demand for tests recently has pushed laboratories to their limit. People are forced to wait days to receive results. How to deal with that?
The clinical laboratory machines for automated data analysis attract with their obvious benefits – process expedition and human error reduction. As stated in the report, the evaluation of the global laboratory automation systems market will be $5.2 billion by 2022.
First and foremost, CV technologies are employed to evaluate blood samples by a taken picture with a view to identifying abnormalities. Other computer vision use cases in healthcare refer to the levels of separated phases of blood, and the quality of centrifuged blood samples ranked and classified by computer vision systems. Worth mentioning also are achievements in automated lab tests concerned with finding meniscus in a vial.
We’re not almighty, but we are at hand with the technology that can.
CONTACT USCompliance and responsibility for results of computer vision medical applications
When it comes to sharing responsibility between the doctor and AI, health workers are a bit skeptical about the issue. Many of them believe that a doctor’s validation of diagnosis is obligatory for patients to be sure that everything is correct.
Another area of concern is the responsibilities between the doctor or hospital and the AI software provider. The latter is expected to provide failure-free running and maintenance in the long run.
And lastly, the participation of doctors is mandatory while developing solutions based on computer vision in the medical industry.
To say unequivocally that one side or another has a larger responsibility, we can’t. In its turn, the intensive spread of medical devices incorporating artificial intelligence in everyday life led to the appropriate response from the FDA.
Look up at the healthcare IoT device usage. We’ve shared best practices for security and our advice.
Their assistance in digital therapeutics prosperity is reflected in approving a number of algorithms covering different medical diagnostic directions. To not be empty, some of them are OsteoDetect by Imagen, IDx-DR by IDx, Accipio Ix by MaxQ AI, AFib algorithm by AliveCor, and others.
If you want to make a compliant solution for the global market, you should check the requirements of the following entities:
- The US Food and Drug Administration
- Health Canada
- The Therapeutic Goods Administration in Australia
- The European Medicines Agency
- The Swiss Agency for Therapeutic Products
- The Japanese Pharmaceuticals and Medical Devices Agency
The non-compliant entities pay civil penalties. Know how to lay down the right basis with HIPAA-compliant chat APIs and SDKs.
Technologies behind computer vision in healthcare applications
The running mechanism behind medical computer vision is models. Models are a set of mathematical formulas that are implemented through frameworks, libraries, and relevant tools.
The technologies behind solutions that allow machines to see like humans with computer vision in medicine are:
Technology | Application |
---|---|
Backend / Core | |
Python | Programming language understandable by humans that allows delivering concise and readable code, easily compatible with other programming languages |
Algorithms | |
OpenCV | Framework fitted for cases with increased computational efficiency which image processing with diverse situations is |
NumPy | Library with high-level math functions, random number generators, best for working with multidimensional arrays |
Tensorflow | Google-backed collection of workflows for neural network models development and training to achieve the quality of human perception |
PyTorch | Open-source framework based on the Torch library, applied for computer vision and natural language processing applications |
API | |
Flask | Python-based micro framework for building web interfaces and APIs in deployment image classification models |
BentoML | Open-source library for high-performance ML model inference, used for building production API endpoints for the ML models |
Cortex | Open-source platform for deployment trained ML models |
Apart from custom-developed ML models, which are quite common in machine learning for biomedical imaging applications today, some open models are already trained and are at arm’s length of research. Since both clients and Aimprosoft are keen to get the qualitative solution more efficiently, it is justified to use available open data sets created to cover popular healthcare and computer vision tasks.
Aimprosoft’s capabilities in implementing machine learning in healthcare
Main article: Machine Learning in Healthcare: From Not-For-Everyone Treatment to Mass-market
Today CV reached the level and accuracy and more than earned the place of a full partner next to the human doctor.
We at Aimprosoft happen to be the ones who are involved in several projects, among which:
- Medication inventory management by means of stereo images;
- Intake monitoring for patients;
- Recommendation engine based on implicit and explicit feedback;
- Image recognition to limit the access of private data;
- ML-based security system to control access to medical records;
- Image measurer application.
Everyone knows seeing is believing. Computer vision is high-tech assistance for conventional doctors that helps save people’s lives. More and more often, healthcare providers tend to set up modern equipment with intensive software support, whereas machine learning for biomedical imaging applications is far from last. Contact us if you’re on the way to making a reliable virtual assistant for doctors. We can consult you and set up a team of data scientists and ML developers to create software that acts like a human.
The future of computer vision in the medical industry
The figures are first: according to Statista, the market size of computer vision in healthcare is expected to reach $50.97 billion by 2030.
The future of imaging in healthcare holds immense potential for transforming healthcare delivery, diagnostics, and patient outcomes in the medical industry. Computer vision project ideas are wide enough to move forward in the industry.
Over the past decade, advances in deep structural learning and artificial intelligence have increased the accuracy of object detection and classification accuracy from 50% to 99%. This is the future that has arrived today.
Computer vision can help visually impaired individuals map their surroundings and provide an alternative to guide dogs. So, the future of it is in more autonomous navigation.
Let’s have a look at Google’s DeepMind artificial intelligence algorithms. They can accurately analyze medical images such as X-rays, CT scans, and retinal images and identify diabetic retinopathy, facilitating early intervention for treatment.
Another prominent future-proof example of a vision computer in the medical field refers to scientists at MIT. They believe there is one way to improve computer vision – to teach the artificial neural networks they rely on to intentionally mimic the way the brain’s biological neural network processes visual images. Jim DiCarlo, a Professor of Systems and Computational Neuroscience at MIT believes that future computer vision could be improved by incorporating specific brain-like features into models.
Lots was said. And finally, there is a list of some more examples where technology will cope better than a human:
- Natural language processing in medicine;
- Personalized medical recommendations;
- Treatment recommendations for physicians;
- Clinical trial guidelines;
- Monitoring patients instead of nurses.
Advantages and challenges of using computer vision in healthcare
There’s no one cure-all. Any technology, be it a computer in the medical field or over-the-counter medicines, meaning a platform Vision Healthcare, has pros and cons. Let’s see what positive this technology in the medical field brings.
Benefits
Improved diagnostics of diseases
Computer vision algorithms can accurately analyze X-rays, CT scans, and MRIs. And can, in doing so, reveal subtle patterns, anomalies, and signs of disease that humans may overlook.
Real-time assistance in surgery
Computer vision technologies provide real-time guidance and assistance in surgical procedures. Specialists use augmented reality (AR) overlay and 3D reconstructions to improve the accuracy of surgical procedures.
Remote monitoring of patients
A patient’s condition can be diagnosed remotely by analyzing visual data such as video images or pictures. In addition to providing more accurate diagnoses, medical professionals can provide counseling in underserved areas.
Automation of routine tasks
Analyzing medical images, extracting data from electronic health records (EHRs), and administrative processes don’t necessarily have to be performed by physicians. The medical computer software can handle these, leaving physicians time for patients.
Faster medical research
To speed up medical research and drug development, computer vision algorithms can analyze vast amounts of medical imaging data, genetic information, and clinical records.
Challenges
Visionary technology developed for the computer in medicine has drawbacks as it is normal. The most evident are listed below.
Data quality and availability
Computer vision model training requires high-quality and diverse datasets of actual patients. However, the difficulty lies in respecting the confidentiality of the data when used with patient consent, as well as the fragmentation of the data itself and its limited availability.
Ethical and legal aspects
Patient privacy, data security, and algorithm errors touch on the ethical side of the issue. And violations or bad faith enforcement of, for example, the HIPAA law, can be a stumbling block to the development of the technology.
Integration and interoperability
Integrating computer vision systems with existing healthcare infrastructure computer vision APi, such as EHRs or medical devices, may not achieve interoperability. And with it, implementing and benefiting from the technology is possible.
Validation and regulation
Among other things, these mechanisms need rigorous validation processes, standards, and regulatory frameworks. Only then can we expect a reliable evaluation and approval of computer vision systems for clinical applications.
FAQ
What are the disadvantages of computer vision in healthcare?
Even though CV has stood up well, it can’t do without the final approval of diagnosis by a human. Parameters for data set training are set by humans, and there is a place for biases still. Systems need regular monitoring to prevent technical glitches or breakdowns.
Do we need special medical devices to implement computer vision in our treatment process?
In addition to software algorithms, it is necessary to use hardware systems such as cameras or IoT sensors.
Can you implement a computer vision into a current application?
It isn’t an issue for us, but the final solution depends on the type of the current application. For instance, if we use a С-based programming language, we can use the OpenCV library to integrate a model. Alternatively, we can implement an API to work with the ML solution in general.
Which model is best for computer vision?
There are a lot of tasks related to computer vision, such as object detection, semantic segmentation, image classification, etc. Each task has a set of datasets used to evaluate the accuracy of a model. Therefore, different models can achieve state-of-art performance on a different dataset. For instance, the best model for real-time object detection on the COCO dataset is YOLOR. In short, there is no one best model for computer vision, but there are best models for certain CV tasks.
What are the key outcomes of computer vision technology in telemedicine?
First and foremost, it is an immediate, accurate medical assistance remotely. Doctors can ‘see’ patients with ‘computer eyes,’ which accelerates primary care by avoiding spending time on trips to the hospital or waiting hours for appointments. Machine vision in healthcare makes care more affordable for many population categories.