AI Strategy and Business Readiness: Interview with Aimprosoft’s AI Expert
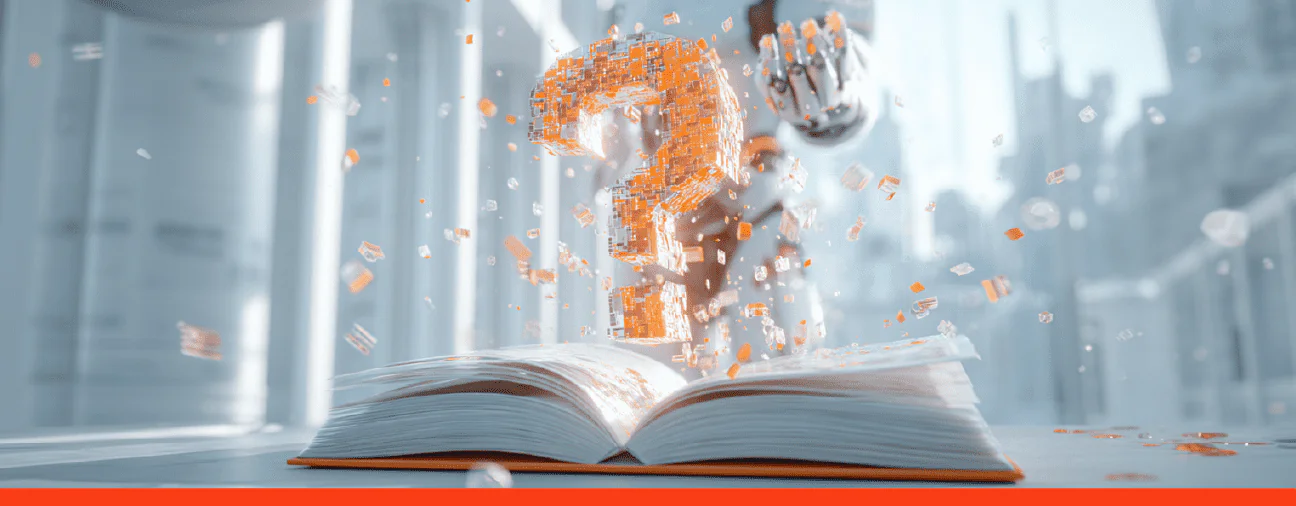
In our conversations with tech leaders about AI-assisted software development, we keep hearing the same tough questions: Is our company ready? How do we set and measure KPIs? What’s the real ROI? These questions impact budgets, timelines, and careers. While AI for software development promises transformative results, many business leaders still see it as a black box — full of potential but opaque in practice.
To address these real-world challenges, we’re sitting down with our AI experts who work directly with these issues. In this first interview, Nick Martynenko, our Head of Development, shares lessons from implementing AI across various projects and developing an effective AI strategy for business.
We’ll cover three critical areas: assessing your organization’s AI readiness, aligning your AI implementation strategy with business goals, and building internal AI capabilities that support lasting success through AI integration in business.
#1 AI Readiness Assessment
How do I know if my company is AI-ready?
Your company’s AI readiness assessment depends on four key areas: people, access to tools, data readiness, and infrastructure compatibility. You can perform this internally or partner with providers of AI technology assessment services for a deeper evaluation. Without that foundation, business AI implementation will struggle to deliver results.
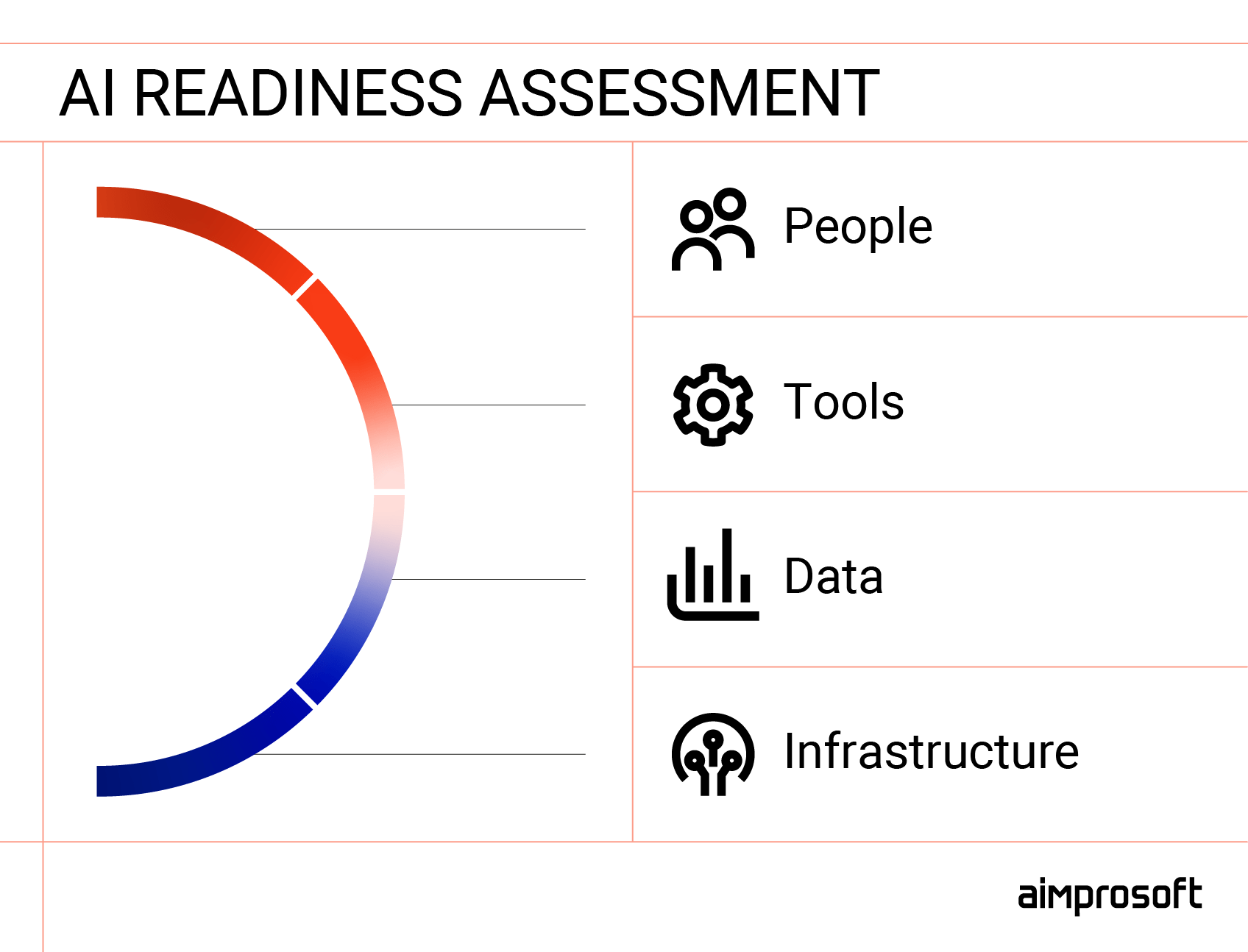
- People and training
Are your employees equipped to work with AI? This includes both their knowledge of AI tools and how actively they use them in their daily work. It’s essential to understand whether they’ve had proper training and whether they feel confident using AI solutions.
- Access to tools
Does your team have the right tools to actually use AI? That means having appropriate subscriptions (e.g., enterprise-level rather than personal), clear access controls, and transparency into how tools are being used across teams. This point is significant at scale as centralized enterprise accounts offer stronger control and reduce risks around tool sprawl or misuse.
- Data readiness
Are your internal data assets prepared for AI processing? If you have a large document repository, those documents need to be standardized and stored in a structured way. Otherwise, AI can’t easily access or interpret your data, and won’t deliver real value.
- Infrastructure readiness
Is your infrastructure prepared for modern AI solutions, including no-code tools and AI agents? Protocols like MCP (Machine Conversation Protocol) are becoming essential for integrating AI with systems like Jira or SharePoint. If your tools support these integrations, you’re well-positioned to move quickly. If not, consider your future needs — AI agents will require proper system access to monitor activities, trigger actions, and perform tasks reliably.
How do I determine if my AI strategy is still relevant 6 months after launch?
Review your original AI strategy framework regularly and be willing to adjust. Make sure your tools are actively supported, legally viable for your region, and aligned with your long-term vision. This means paying attention to how fast AI tech is improving, seeing if the platforms you’ve picked will last, and knowing how much it might cost to switch if a model gets outdated. After all, choosing the wrong tech can cost you more in the end than spending the time to choose wisely from the start.
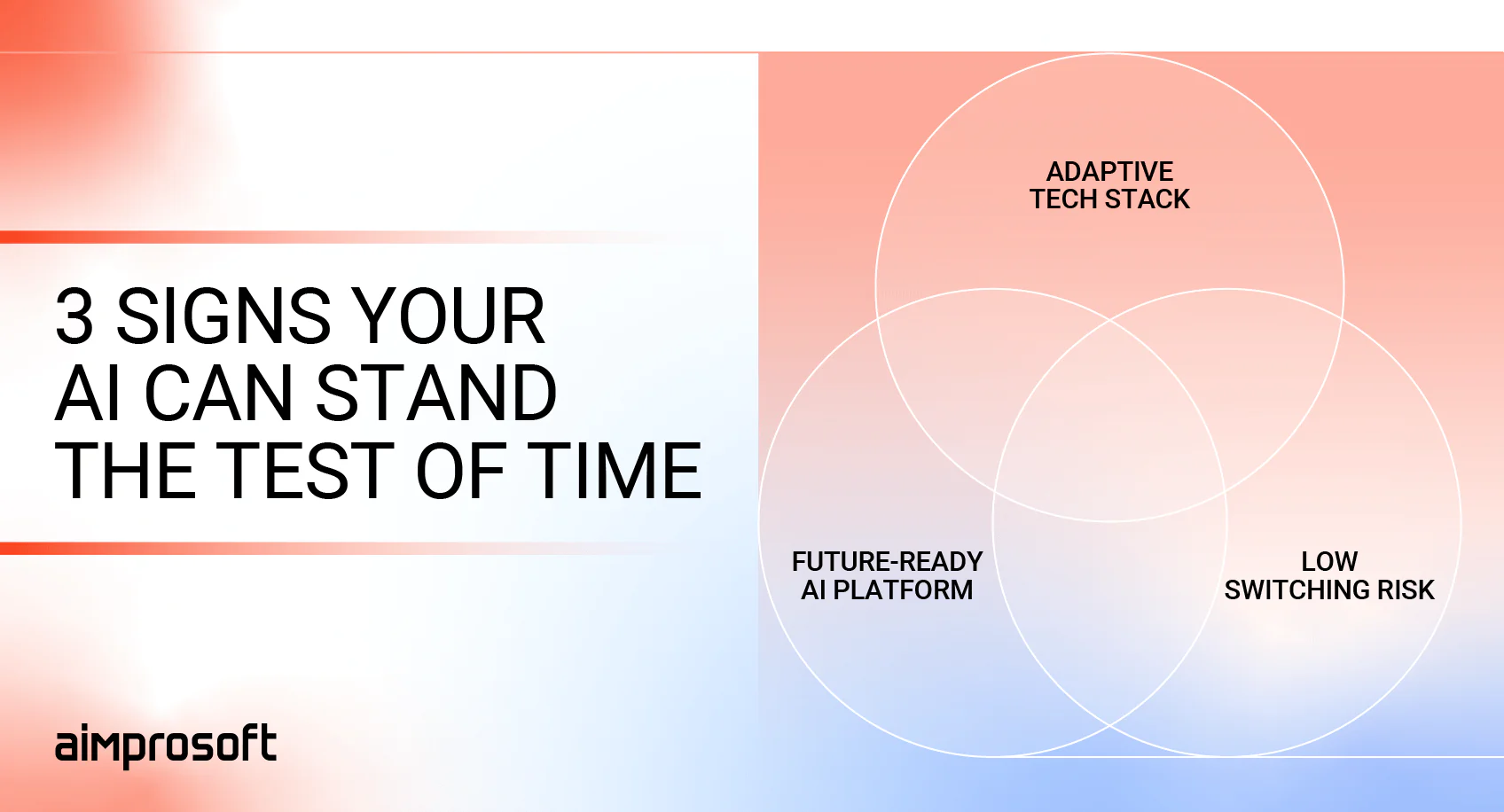
- Track technological evolution
AI is moving at a breakneck pace, similar to other hyped technologies we’ve seen before. Think back to the early days of Java or JavaScript, where a flood of libraries and frameworks made it hard to choose the “right” one. Some tools thrived, others faded. The same applies to today’s AI tools, especially large language models (LLMs). Companies adopting generative AI development services need to carefully assess the maturity and long-term viability of their chosen models and platforms.
- Evaluate your strategic bets
When defining your enterprise AI strategy — or crafting a generative AI strategy — you’re making strategic bets. For example, choosing between AI models, OpenAI, Mistral, DeepSeek, or LLaMA, isn’t just about current performance — it’s about sustainability, legal considerations, and alignment with your region’s regulations. A well-funded model backed by a stable legal environment (like Mistral in the EU) may be more future-proof than one that could face access restrictions due to geopolitical tensions. This is where an AI integration specialist can provide valuable guidance.
- Calculate switching costs
And don’t underestimate the cost of switching. A model might seem promising today, but if it’s replaced or restructured as Google did with AngularJS vs. Angular (completely different under the hood), you might end up rewriting everything from scratch.
What are the biggest failures companies make when adopting AI?
The three most common failures in AI implementation are insufficient employee training, poor data practices, and treating AI as an add-on rather than integrating it into business strategy.
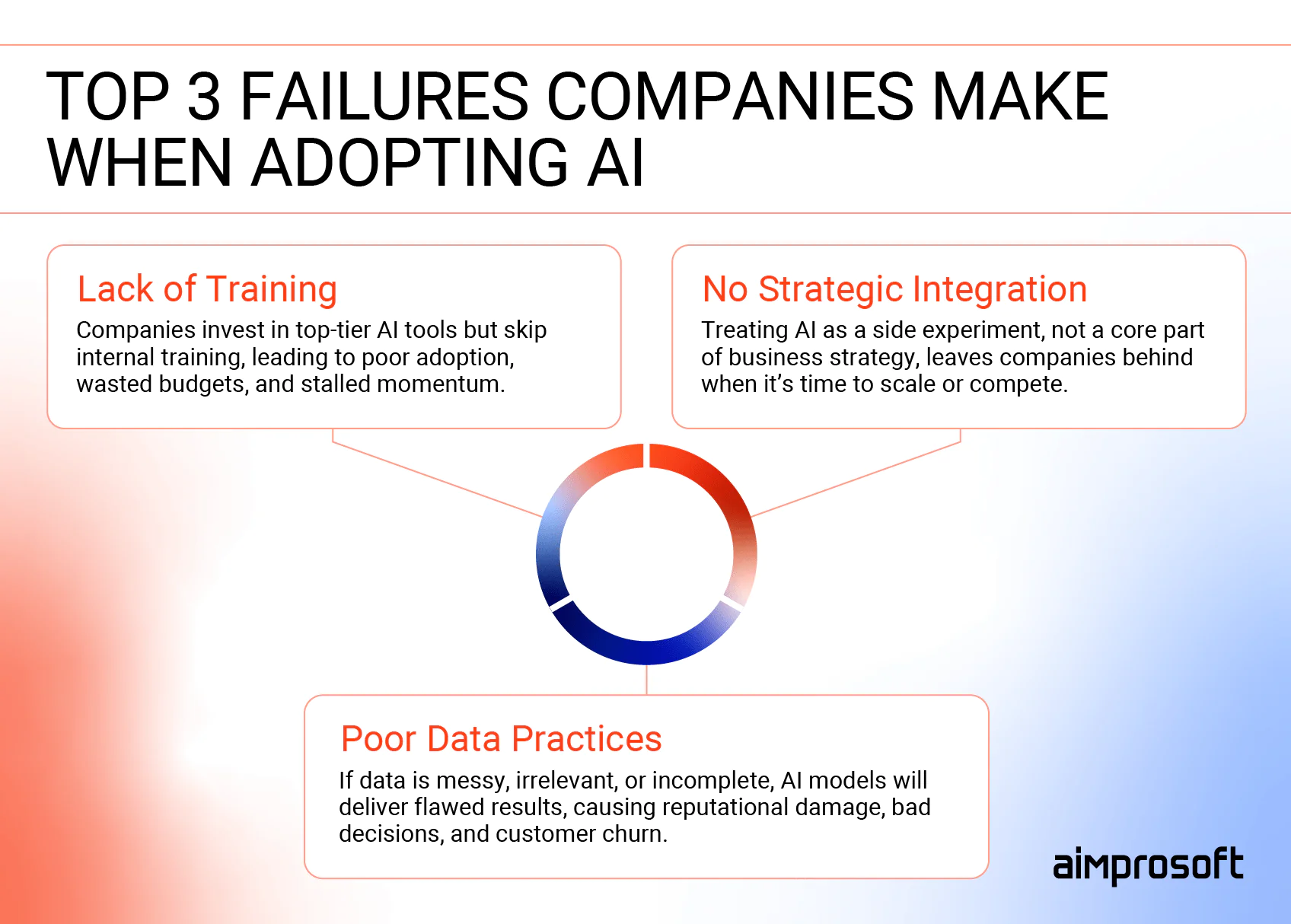
- Lack of training
Some companies jump in headfirst — investing in top-tier servers, tools, and subscriptions — all without preparing the teams that will use AI in software development. The result isn’t just low adoption; it often leads to wasted resources, delayed outcomes, frustrated stakeholders, and, in some cases, loss of internal trust or future funding.
- Overreliance on AI without proper data practices
Others fall into the trap of feeding AI models all available data, expecting magic. But if the data is unstructured, inconsistent, or irrelevant, the output will reflect that — leading to inaccurate insights, flawed decisions, or even automated errors. The consequences can be severe, from reputational damage and regulatory risks to wasted budget and costly rework. In some cases, poor AI output can directly impact customers, leading to a loss of trust and business. An early AI impact assessment helps organizations catch these risks before they escalate, ensuring that AI adoption is based on quality foundations rather than hopeful assumptions.
- No strategic integration of AI into business planning
Perhaps the most dangerous mistake is treating AI as an add-on rather than part of the long-term AI business strategy. If AI isn’t part of your roadmap today, it’s easy to fall behind tomorrow. We’ve seen this before, like during the early days of COVID, when some companies quickly transitioned to digital services while others struggled or failed because they weren’t prepared.
#2 Strategic AI Alignment
How do I align AI product development with long-term company vision and brand values?
Achieving successful AI alignment starts with four essentials: a strong product mindset, a culture of ownership, clear business metrics, and disciplined timeline management. These practices form the foundation for a sustainable AI adoption framework that scales across teams and supports long-term growth.
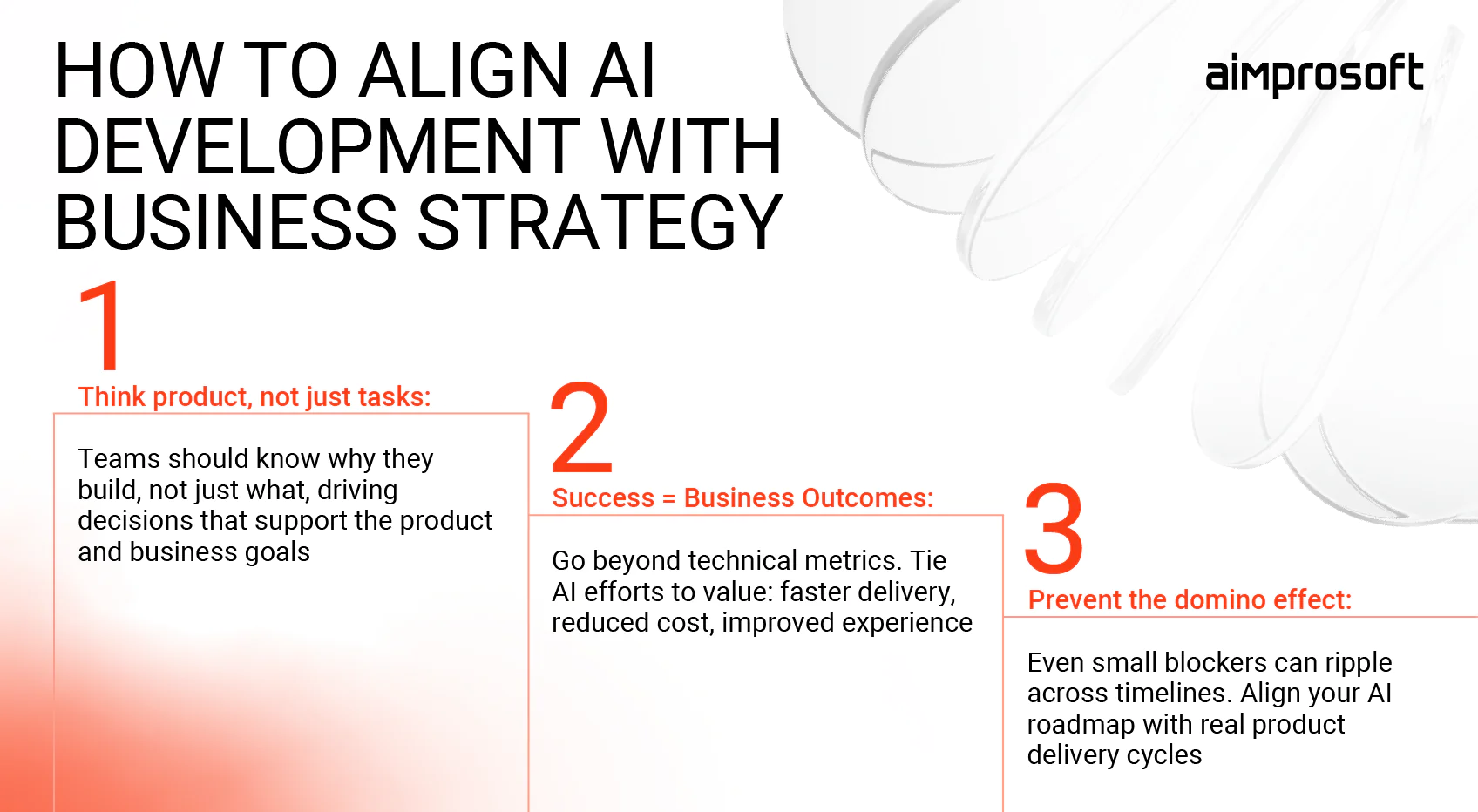
- Develop a product mindset
Individuals who don’t just follow instructions but understand the why behind every feature, automation, or optimization are the key to a company’s success. They ask not only what we are building but why we are building it, how it improves the product, and what value it brings to the user or business. When you have this mindset across the company, people start making smarter decisions. They suggest tools based on business goals, challenge unnecessary complexity, and work toward outcomes that align with your brand and mission — not just tasks. This approach is essential for effective AI in product development.
- Establish clear success metrics
To keep AI efforts aligned with your brand and strategy, you need more than just technical KPIs. Define what success actually looks like — whether it’s improved customer satisfaction, faster delivery, reduced manual effort, or measurable cost savings. These metrics not only guide prioritization but also help stakeholders understand the real business value of AI beyond technical performance. Plus, when metrics are tied to outcomes, not just activity, it becomes easier to evaluate what’s working and what isn’t. This is a key component of AI strategy development.
- Prevent the domino effect
When people don’t see how their work connects to the bigger picture, even minor delays — like refining a prompt, finalizing copy, or finishing a design — can trigger a ripple effect across the entire product. A missed deadline in one area can push back development, delay AI model deployment, stall marketing campaigns, or cause the product to miss its market window. If your AI implementation strategy isn’t clearly tied to delivery timelines and downstream business processes, you risk falling behind — even if the technology itself is solid.
How can I measure the maturity of my company’s AI adoption beyond standard frameworks?
AI maturity isn’t just about what tech you have. It’s about how you use it. To truly assess it, look at how purposefully AI is applied, how well it fits into daily workflows, how confident employees are with it, the measurable business impact, and whether your AI tools still align with your strategy. A strong AI maturity assessment reveals how people think, work, and create value — not just which tools are in place.
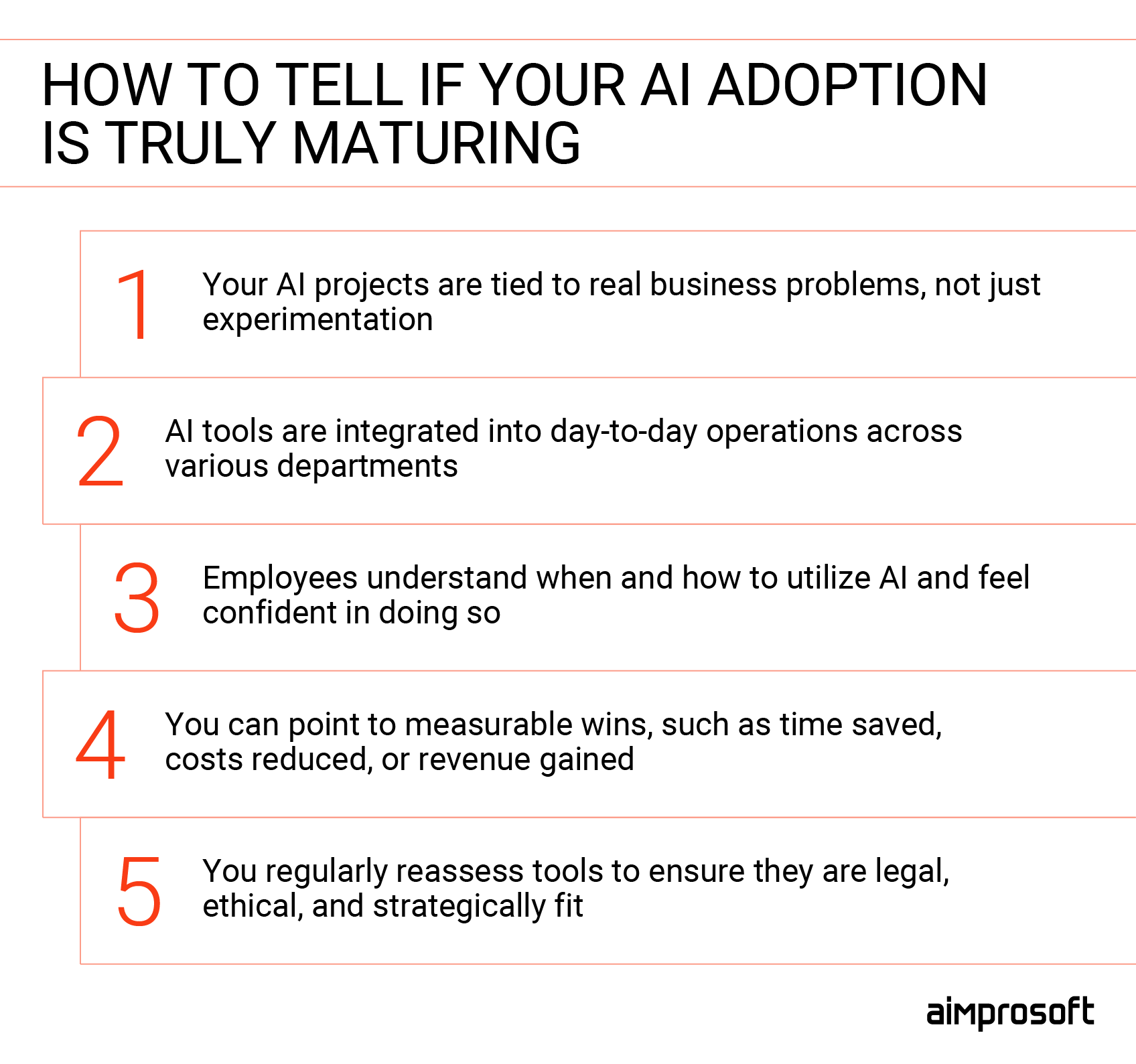
- Use AI with intention, not just curiosity
AI shouldn’t be treated as a one-off experiment. The most effective teams connect AI software development services to real business goals — like improving operations, enhancing customer experience, or reducing manual effort. Curiosity is useful early on, but long-term value comes from solving specific, meaningful problems.
- Assess how deeply AI is embedded into workflows
Look at how deeply AI tools or automation are embedded in everyday operations. Successful AI integration in business means product managers use it to sort priorities, marketers leverage it for insights, and support teams automate common queries. The more naturally AI fits into the way people already work, the more impact it can have.
- Evaluate employee skills and critical thinking around AI
It’s not just about knowing how to use tools like ChatGPT or Copilot. It’s about cultivating a culture where people ask: “Should we use AI here?” and “Is this the right way to do it?” Combining practical skills with critical thinking helps teams spot real opportunities and avoid unnecessary complexity.
- Track the real business impact of AI
It’s easy to celebrate adoption, but the real question is: what has AI-assisted software development actually delivered? Strong teams can point to specific wins, such as time saved, costs lowered, better decisions, or new revenue. If those outcomes aren’t clear, it may be time to rethink how success is being measured.
- Reassess your AI stack as things evolve
AI is changing fast. A model or platform that worked well last year might not be the best fit today. It’s important to check regularly whether your tools still meet legal, ethical, and business needs, not just rely on what was trendy when you started. Partnering with experienced AI strategy consulting firms can help you stay current with these evolving technologies.
#3 Building AI Organizational Capabilities
How do I build an internal AI consulting capability in my organization?
Building an internal AI consulting capability is about systems, culture, and people. Companies often benefit from support by an AI implementation consultant or AI integration consulting partner during the early stages to structure scalable learning and delivery paths. But more importantly, you need people who take ownership, grow continuously, and make decisions with clarity and care.
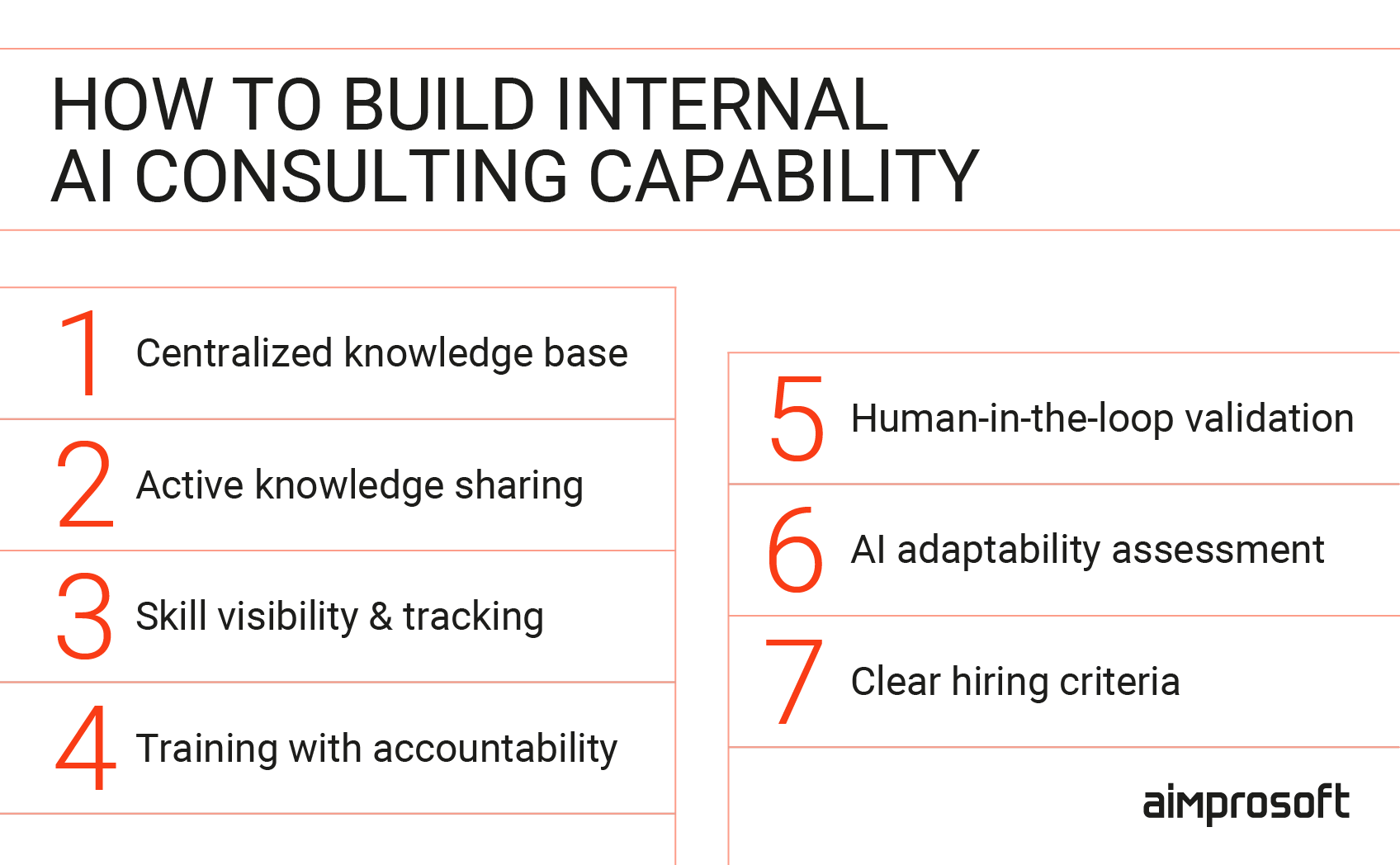
- Establish a reliable knowledge base
Start with accessible resources. That could be internal documentation, curated courses, recorded webinars, shared success cases — anything that helps your team understand how AI augmented development can support your business. Without a shared base of knowledge, your internal consulting efforts will struggle to scale.
- Encourage ongoing knowledge-sharing
Create regular spaces for discussion: team meetups, AI demos, post-mortems, and internal talks. These don’t need to be formal. What’s important is that people learn from one another and see AI wins (and mistakes) in context.
- Define and track skills through a capability matrix
We use a skill matrix inside our Aim Academy to track the knowledge and abilities expected from team members. This step helps us validate who’s ready to advise others, who needs support, and which knowledge gaps we need to close. Over time, this can evolve into more structured metrics, such as test-based evaluations or certification-linked learning paths.
- Balance training and accountability
Training is only one part of the equation. People need to know that their outputs — especially when AI is involved — carry real consequences. AI can hallucinate (generate incorrect information). If you accept and approve its output, you’re still responsible. That’s why we stress both product mindset and accountability as part of the implementation of AI. Everyone should understand the value of their contribution and the impact of their decisions.
- Validate with human oversight, always
Human-in-the-loop validation (where a person reviews and approves AI outputs before implementation) is non-negotiable for effective generative AI implementation. Whether it’s generated code or content, someone must check if it’s correct, helpful, and aligned with the company’s standards. This loop of review and refinement is what prevents AI from becoming a liability.
- Be honest about fit
Some people will embrace AI. Others won’t. That’s a leadership challenge: help those who are open to grow and respectfully part ways with those who can’t or won’t adapt. It doesn’t mean blaming individuals — it means evaluating performance fairly and offering clear feedback. If improvement doesn’t come, it’s okay to make space for someone who’s better aligned with where your company is going.
- Support recruiters and L&D with clear criteria
As AI roles evolve, recruiters and learning teams need your help to define what “AI-ready” actually looks like. Especially when hiring juniors or interns, be specific about what skills you want them to develop — and give them the tools to get there.
What’s the best way to structure an AI Center of Excellence?
An effective AI Center of Excellence (CoE) requires realistic expectations, clear skill benchmarks, strategic purpose, cross-departmental support, and alignment with both company strategy and individual development. Partnering with AI integration services teams can further accelerate setup by advising on scalable architectures and reusable frameworks.
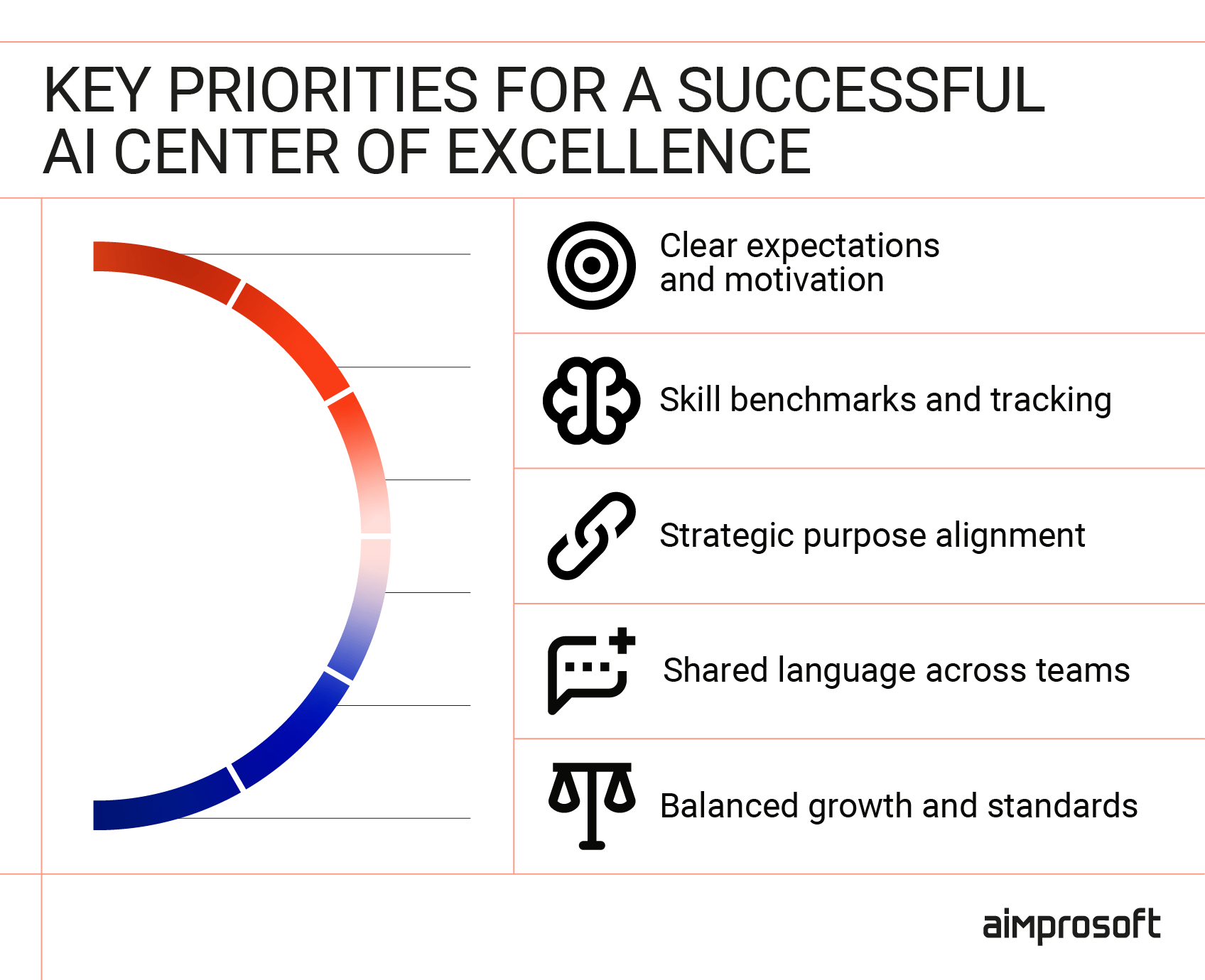
- Set realistic expectations and incentives
Don’t assume people will naturally engage just because the CoE exists. Participation often requires extra effort beyond core responsibilities, so think through what motivates involvement. These can be сompany-wide policies that prioritize upskilling. Recognition systems or bonuses tied to CoE contributions. Exclusive access to training, pilot projects, or external certifications. If there’s no value in participating, people won’t opt in.
- Define skill benchmarks and track progress
Create skill matrices that map to your strategic goals. For instance, if your CoE aims to grow in AI-assisted software development, start by defining what an ideal AI-ready developer or analyst looks like. Then, assess your team against those profiles. You might find someone labeled “senior” doesn’t actually meet your internal benchmarks. Use this to set individual growth paths and measure progress over time. If the average skill level increases across the company, your CoE is doing its job.
- Understand the “why” behind the CoE
This isn’t just a Learning and Development (L&D) initiative — it’s a strategic differentiator. If you’re a service company, offering well-prepared talent to clients is your brand. Sending underprepared candidates to clients damages both trust and reputation. A CoE helps ensure you’re not just filling roles but putting forward people who’ve been vetted, trained, and aligned with current company priorities.
- Support other departments with shared language and clarity
With a skill matrix in place, your sales team can confidently answer client questions about your team’s capabilities. Your recruiters know what to look for. Your delivery managers understand what to expect from team members. Everyone operates with the same definitions and expectations — no more apples-to-bananas comparisons.
- Balance strategic direction with individual development
The CoE should reflect where your company is going. If your strategic focus is shifting toward AI agents or ML infrastructure, your internal learning and hiring priorities need to shift, too. At the same time, you must stay grounded in people’s development. Use skill assessments to identify who’s ready to grow and who might not be a long-term fit. And be prepared: if someone repeatedly fails to meet standards despite feedback and support, it may be time to part ways. The CoE exists to raise the bar — not lower it to accommodate everyone.
What organizational changes are needed to support AI at scale?
Scaling AI requires organizational changes across team structure, governance roles, performance metrics, education programs, knowledge management, and experimentation frameworks.
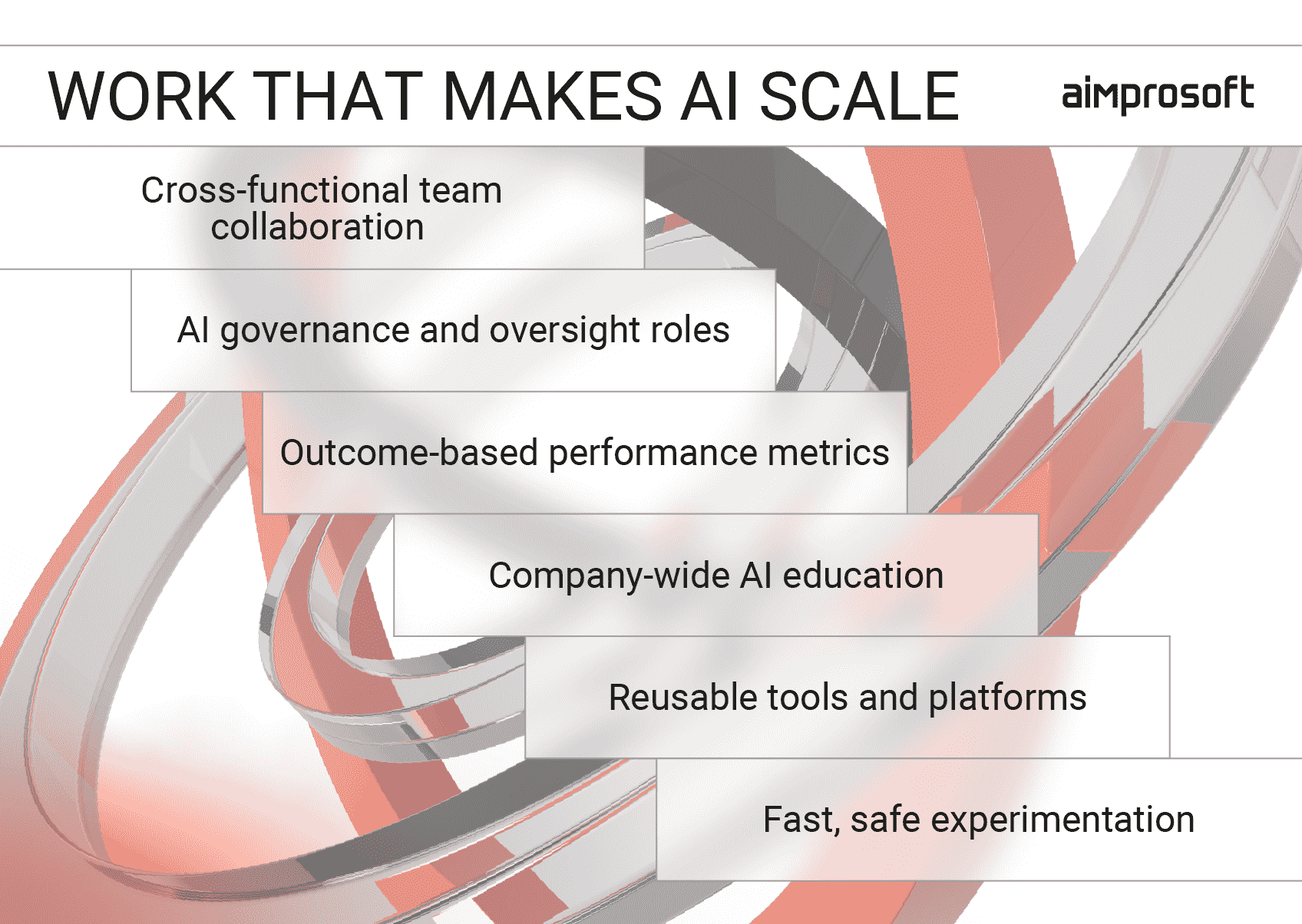
- Shift from siloed functions to cross-functional collaboration
AI initiatives thrive when business, data, and engineering teams work together. That means breaking down silos between departments and embedding AI specialists — like data scientists, ML engineers, or AI consultants — into product, operations, and marketing teams. You need shared goals, not parallel efforts.
- Introduce roles focused on AI governance and quality
As AI use grows, it becomes essential to have a clear structure around how it’s applied across the organization. This means introducing roles like AI product owners, who ensure development efforts stay aligned with business goals, and AI governance leads, who are responsible for overseeing ethics, compliance, and responsible usage. You’ll also need human-in-the-loop roles — people tasked with reviewing and validating AI outputs, especially in areas where risk is high. These aren’t just buzzwords; they’re critical to making sure AI decisions are trusted, explainable, and auditable.
- Adapt performance metrics and incentives
Traditional KPIs may not capture the impact of AI projects. Teams working on automation, predictive models, or agent-based tools need metrics tied to outcomes like efficiency gains, decision accuracy, or time saved — not just code deployed or features launched.
- Build internal AI education programs
To avoid creating two classes of employees — those who “get AI” and those who don’t — organizations need to invest in company-wide enablement. This means offering AI literacy training for non-technical roles, providing advanced learning paths and certifications for engineers, and encouraging internal sharing of successful use cases to build momentum and inspire others. When everyone has a baseline understanding of AI assessment methods and access to growth opportunities, adoption becomes more inclusive and sustainable.
- Create a scalable knowledge and tooling ecosystem
You’ll need standardized tools, reusable components, and a shared knowledge base to avoid duplication and shadow projects. This is where internal platforms or AI Centers of Excellence (CoEs) come in. Without them, every team ends up reinventing the wheel — and scaling stalls.
- Enable fast, responsible experimentation
Finally, the scale doesn’t mean rigidity. You’ll need lightweight processes for experimentation: safe sandboxes, fast procurement paths for new tools, and clear guidelines on what can be tested, where, and by whom.
What’s the right team structure for AI product development in a mid-sized company?
The right AI team structure depends on whether you’re building AI-powered products (requiring specialized AI roles) or using AI tools to enhance traditional development (working with standard cross-functional teams).
For AI-assisted software development:
For teams working at the intersection of AI and software development, traditional cross-functional structures often remain effective.
- Product Manager (PM)
- Business Analyst (BA)
- Solution Architect
- Frontend and Backend Developers
- DevOps Engineer
- QA Engineers (increasingly focused on automation)
Depending on the product size, roles like PM or BA can be shared across projects part-time. The key here is that your team uses AI to accelerate the development process.
For AI-native or AI-heavy products:
However, when it comes to AI-native or AI-heavy products — such as agent-based platforms, generative AI for software development, or applications powered by large language models — the team setup looks quite different:
- Solution Architect: to design how AI components (agents, models, APIs) interact with the rest of the system
- Data Analyst or Data Scientist: to assess data quality, movement, transformation, and value
- AI/ML Engineer: If you’re fine-tuning models or integrating third-party AI services
Around this core, you’ll build out your broader dev team: frontend and backend engineers, DevOps to handle complex and frequent deployments, and QA engineers focused on automated testing within your CI/CD pipelines.